Chapter 16 – Natural Language Processing with RNNs and Attention**#
This notebook contains all the sample code in chapter 16.
Setup#
First, let’s import a few common modules, ensure MatplotLib plots figures inline and prepare a function to save the figures. We also check that Python 3.5 or later is installed (although Python 2.x may work, it is deprecated so we strongly recommend you use Python 3 instead), as well as Scikit-Learn ≥0.20 and TensorFlow ≥2.0.
# Python ≥3.5 is required
import sys
assert sys.version_info >= (3, 5)
# Is this notebook running on Colab or Kaggle?
IS_COLAB = "google.colab" in sys.modules
IS_KAGGLE = "kaggle_secrets" in sys.modules
if IS_COLAB:
%pip install -q -U tensorflow-addons
%pip install -q -U transformers
# Scikit-Learn ≥0.20 is required
import sklearn
assert sklearn.__version__ >= "0.20"
# TensorFlow ≥2.0 is required
import tensorflow as tf
from tensorflow import keras
assert tf.__version__ >= "2.0"
if not tf.config.list_physical_devices('GPU'):
print("No GPU was detected. LSTMs and CNNs can be very slow without a GPU.")
if IS_COLAB:
print("Go to Runtime > Change runtime and select a GPU hardware accelerator.")
if IS_KAGGLE:
print("Go to Settings > Accelerator and select GPU.")
# Common imports
import numpy as np
import os
# to make this notebook's output stable across runs
np.random.seed(42)
tf.random.set_seed(42)
# To plot pretty figures
%matplotlib inline
import matplotlib as mpl
import matplotlib.pyplot as plt
mpl.rc('axes', labelsize=14)
mpl.rc('xtick', labelsize=12)
mpl.rc('ytick', labelsize=12)
# Where to save the figures
PROJECT_ROOT_DIR = "."
CHAPTER_ID = "nlp"
IMAGES_PATH = os.path.join(PROJECT_ROOT_DIR, "images", CHAPTER_ID)
os.makedirs(IMAGES_PATH, exist_ok=True)
def save_fig(fig_id, tight_layout=True, fig_extension="png", resolution=300):
path = os.path.join(IMAGES_PATH, fig_id + "." + fig_extension)
print("Saving figure", fig_id)
if tight_layout:
plt.tight_layout()
plt.savefig(path, format=fig_extension, dpi=resolution)
No GPU was detected. LSTMs and CNNs can be very slow without a GPU.
Char-RNN#
Splitting a sequence into batches of shuffled windows#
For example, let’s split the sequence 0 to 14 into windows of length 5, each shifted by 2 (e.g.,[0, 1, 2, 3, 4]
, [2, 3, 4, 5, 6]
, etc.), then shuffle them, and split them into inputs (the first 4 steps) and targets (the last 4 steps) (e.g., [2, 3, 4, 5, 6]
would be split into [[2, 3, 4, 5], [3, 4, 5, 6]]
), then create batches of 3 such input/target pairs:
np.random.seed(42)
tf.random.set_seed(42)
n_steps = 5
dataset = tf.data.Dataset.from_tensor_slices(tf.range(15))
dataset = dataset.window(n_steps, shift=2, drop_remainder=True)
dataset = dataset.flat_map(lambda window: window.batch(n_steps))
dataset = dataset.shuffle(10).map(lambda window: (window[:-1], window[1:]))
dataset = dataset.batch(3).prefetch(1)
for index, (X_batch, Y_batch) in enumerate(dataset):
print("_" * 20, "Batch", index, "\nX_batch")
print(X_batch.numpy())
print("=" * 5, "\nY_batch")
print(Y_batch.numpy())
____________________ Batch 0
X_batch
[[6 7 8 9]
[2 3 4 5]
[4 5 6 7]]
=====
Y_batch
[[ 7 8 9 10]
[ 3 4 5 6]
[ 5 6 7 8]]
____________________ Batch 1
X_batch
[[ 0 1 2 3]
[ 8 9 10 11]
[10 11 12 13]]
=====
Y_batch
[[ 1 2 3 4]
[ 9 10 11 12]
[11 12 13 14]]
Loading the Data and Preparing the Dataset#
shakespeare_url = "https://raw.githubusercontent.com/karpathy/char-rnn/master/data/tinyshakespeare/input.txt"
filepath = keras.utils.get_file("shakespeare.txt", shakespeare_url)
with open(filepath) as f:
shakespeare_text = f.read()
Downloading data from https://raw.githubusercontent.com/karpathy/char-rnn/master/data/tinyshakespeare/input.txt
1122304/1115394 [==============================] - 0s 0us/step
print(shakespeare_text[:148])
First Citizen:
Before we proceed any further, hear me speak.
All:
Speak, speak.
First Citizen:
You are all resolved rather to die than to famish?
"".join(sorted(set(shakespeare_text.lower())))
"\n !$&',-.3:;?abcdefghijklmnopqrstuvwxyz"
tokenizer = keras.preprocessing.text.Tokenizer(char_level=True)
tokenizer.fit_on_texts(shakespeare_text)
tokenizer.texts_to_sequences(["First"])
[[20, 6, 9, 8, 3]]
tokenizer.sequences_to_texts([[20, 6, 9, 8, 3]])
['f i r s t']
max_id = len(tokenizer.word_index) # number of distinct characters
dataset_size = tokenizer.document_count # total number of characters
[encoded] = np.array(tokenizer.texts_to_sequences([shakespeare_text])) - 1
train_size = dataset_size * 90 // 100
dataset = tf.data.Dataset.from_tensor_slices(encoded[:train_size])
Note: in previous versions of this code, we used dataset.repeat()
now to make the dataset “infinite”, and later in the notebook we set the steps_per_epoch
argument when calling the model.fit()
method. This was needed to work around some TensorFlow bugs. However, since these bugs have now been fixed, we can simplify the code: no need for dataset.repeat()
or steps_per_epoch
anymore.
n_steps = 100
window_length = n_steps + 1 # target = input shifted 1 character ahead
dataset = dataset.window(window_length, shift=1, drop_remainder=True)
dataset = dataset.flat_map(lambda window: window.batch(window_length))
np.random.seed(42)
tf.random.set_seed(42)
batch_size = 32
dataset = dataset.shuffle(10000).batch(batch_size)
dataset = dataset.map(lambda windows: (windows[:, :-1], windows[:, 1:]))
dataset = dataset.map(
lambda X_batch, Y_batch: (tf.one_hot(X_batch, depth=max_id), Y_batch))
dataset = dataset.prefetch(1)
for X_batch, Y_batch in dataset.take(1):
print(X_batch.shape, Y_batch.shape)
(32, 100, 39) (32, 100)
Creating and Training the Model#
Warning: the following code may take up to 24 hours to run, depending on your hardware. If you use a GPU, it may take just 1 or 2 hours, or less.
Note: the GRU
class will only use the GPU (if you have one) when using the default values for the following arguments: activation
, recurrent_activation
, recurrent_dropout
, unroll
, use_bias
and reset_after
. This is why I commented out recurrent_dropout=0.2
(compared to the book).
model = keras.models.Sequential([
keras.layers.GRU(128, return_sequences=True, input_shape=[None, max_id],
#dropout=0.2, recurrent_dropout=0.2),
dropout=0.2),
keras.layers.GRU(128, return_sequences=True,
#dropout=0.2, recurrent_dropout=0.2),
dropout=0.2),
keras.layers.TimeDistributed(keras.layers.Dense(max_id,
activation="softmax"))
])
model.compile(loss="sparse_categorical_crossentropy", optimizer="adam")
history = model.fit(dataset, epochs=10)
Epoch 1/10
31368/31368 [==============================] - 7150s 228ms/step - loss: 1.4671
Epoch 2/10
31368/31368 [==============================] - 7094s 226ms/step - loss: 1.3614
Epoch 3/10
31368/31368 [==============================] - 7063s 225ms/step - loss: 1.3404
Epoch 4/10
31368/31368 [==============================] - 7039s 224ms/step - loss: 1.3311
Epoch 5/10
31368/31368 [==============================] - 7056s 225ms/step - loss: 1.3256
Epoch 6/10
31368/31368 [==============================] - 7049s 225ms/step - loss: 1.3209
Epoch 7/10
31368/31368 [==============================] - 7068s 225ms/step - loss: 1.3166
Epoch 8/10
31368/31368 [==============================] - 7030s 224ms/step - loss: 1.3138
Epoch 9/10
31368/31368 [==============================] - 7061s 225ms/step - loss: 1.3120
Epoch 10/10
31368/31368 [==============================] - 7177s 229ms/step - loss: 1.3105
Using the Model to Generate Text#
def preprocess(texts):
X = np.array(tokenizer.texts_to_sequences(texts)) - 1
return tf.one_hot(X, max_id)
Warning: the predict_classes()
method is deprecated. Instead, we must use np.argmax(model(X_new), axis=-1)
.
X_new = preprocess(["How are yo"])
#Y_pred = model.predict_classes(X_new)
Y_pred = np.argmax(model(X_new), axis=-1)
tokenizer.sequences_to_texts(Y_pred + 1)[0][-1] # 1st sentence, last char
'u'
tf.random.set_seed(42)
tf.random.categorical([[np.log(0.5), np.log(0.4), np.log(0.1)]], num_samples=40).numpy()
array([[0, 1, 0, 2, 1, 0, 0, 1, 0, 0, 1, 1, 0, 1, 1, 1, 0, 2, 1, 0, 2, 1,
0, 1, 2, 1, 1, 1, 2, 0, 0, 1, 0, 0, 1, 0, 1, 0, 0, 2]])
def next_char(text, temperature=1):
X_new = preprocess([text])
y_proba = model(X_new)[0, -1:, :]
rescaled_logits = tf.math.log(y_proba) / temperature
char_id = tf.random.categorical(rescaled_logits, num_samples=1) + 1
return tokenizer.sequences_to_texts(char_id.numpy())[0]
tf.random.set_seed(42)
next_char("How are yo", temperature=1)
'u'
def complete_text(text, n_chars=50, temperature=1):
for _ in range(n_chars):
text += next_char(text, temperature)
return text
tf.random.set_seed(42)
print(complete_text("t", temperature=0.2))
the belly the charges of the other words
and belly
print(complete_text("t", temperature=1))
thing! they know't.
biondello:
for you are the own
print(complete_text("t", temperature=2))
th no cyty
use ffor was firive this toighingaber; b
Stateful RNN#
tf.random.set_seed(42)
dataset = tf.data.Dataset.from_tensor_slices(encoded[:train_size])
dataset = dataset.window(window_length, shift=n_steps, drop_remainder=True)
dataset = dataset.flat_map(lambda window: window.batch(window_length))
dataset = dataset.batch(1)
dataset = dataset.map(lambda windows: (windows[:, :-1], windows[:, 1:]))
dataset = dataset.map(
lambda X_batch, Y_batch: (tf.one_hot(X_batch, depth=max_id), Y_batch))
dataset = dataset.prefetch(1)
batch_size = 32
encoded_parts = np.array_split(encoded[:train_size], batch_size)
datasets = []
for encoded_part in encoded_parts:
dataset = tf.data.Dataset.from_tensor_slices(encoded_part)
dataset = dataset.window(window_length, shift=n_steps, drop_remainder=True)
dataset = dataset.flat_map(lambda window: window.batch(window_length))
datasets.append(dataset)
dataset = tf.data.Dataset.zip(tuple(datasets)).map(lambda *windows: tf.stack(windows))
dataset = dataset.map(lambda windows: (windows[:, :-1], windows[:, 1:]))
dataset = dataset.map(
lambda X_batch, Y_batch: (tf.one_hot(X_batch, depth=max_id), Y_batch))
dataset = dataset.prefetch(1)
Note: once again, I commented out recurrent_dropout=0.2
(compared to the book) so you can get GPU acceleration (if you have one).
model = keras.models.Sequential([
keras.layers.GRU(128, return_sequences=True, stateful=True,
#dropout=0.2, recurrent_dropout=0.2,
dropout=0.2,
batch_input_shape=[batch_size, None, max_id]),
keras.layers.GRU(128, return_sequences=True, stateful=True,
#dropout=0.2, recurrent_dropout=0.2),
dropout=0.2),
keras.layers.TimeDistributed(keras.layers.Dense(max_id,
activation="softmax"))
])
class ResetStatesCallback(keras.callbacks.Callback):
def on_epoch_begin(self, epoch, logs):
self.model.reset_states()
model.compile(loss="sparse_categorical_crossentropy", optimizer="adam")
history = model.fit(dataset, epochs=50,
callbacks=[ResetStatesCallback()])
Epoch 1/50
313/313 [==============================] - 62s 198ms/step - loss: 2.6189
Epoch 2/50
313/313 [==============================] - 58s 187ms/step - loss: 2.2091
Epoch 3/50
313/313 [==============================] - 56s 178ms/step - loss: 2.0775
Epoch 4/50
313/313 [==============================] - 56s 179ms/step - loss: 2.4689
Epoch 5/50
313/313 [==============================] - 56s 179ms/step - loss: 2.3274
Epoch 6/50
313/313 [==============================] - 57s 183ms/step - loss: 2.1412
Epoch 7/50
313/313 [==============================] - 57s 183ms/step - loss: 2.0748
Epoch 8/50
313/313 [==============================] - 56s 179ms/step - loss: 1.9850
Epoch 9/50
313/313 [==============================] - 56s 179ms/step - loss: 1.9465
Epoch 10/50
313/313 [==============================] - 56s 179ms/step - loss: 1.8995
Epoch 11/50
313/313 [==============================] - 57s 182ms/step - loss: 1.8576
Epoch 12/50
313/313 [==============================] - 56s 179ms/step - loss: 1.8510
Epoch 13/50
313/313 [==============================] - 57s 184ms/step - loss: 1.8038
Epoch 14/50
313/313 [==============================] - 56s 178ms/step - loss: 1.7867
Epoch 15/50
313/313 [==============================] - 56s 180ms/step - loss: 1.7635
Epoch 16/50
313/313 [==============================] - 56s 179ms/step - loss: 1.7270
Epoch 17/50
313/313 [==============================] - 58s 184ms/step - loss: 1.7097
<<31 more lines>>
313/313 [==============================] - 58s 185ms/step - loss: 1.5998
Epoch 34/50
313/313 [==============================] - 58s 184ms/step - loss: 1.5954
Epoch 35/50
313/313 [==============================] - 58s 185ms/step - loss: 1.5944
Epoch 36/50
313/313 [==============================] - 57s 183ms/step - loss: 1.5902
Epoch 37/50
313/313 [==============================] - 57s 183ms/step - loss: 1.5893
Epoch 38/50
313/313 [==============================] - 59s 187ms/step - loss: 1.5845
Epoch 39/50
313/313 [==============================] - 57s 183ms/step - loss: 1.5821
Epoch 40/50
313/313 [==============================] - 59s 187ms/step - loss: 1.5798
Epoch 41/50
313/313 [==============================] - 57s 181ms/step - loss: 1.5794
Epoch 42/50
313/313 [==============================] - 57s 182ms/step - loss: 1.5774
Epoch 43/50
313/313 [==============================] - 57s 182ms/step - loss: 1.5755
Epoch 44/50
313/313 [==============================] - 58s 186ms/step - loss: 1.5735
Epoch 45/50
313/313 [==============================] - 58s 186ms/step - loss: 1.5714
Epoch 46/50
313/313 [==============================] - 57s 181ms/step - loss: 1.5686
Epoch 47/50
313/313 [==============================] - 57s 181ms/step - loss: 1.5675
Epoch 48/50
313/313 [==============================] - 56s 180ms/step - loss: 1.5657
Epoch 49/50
313/313 [==============================] - 58s 185ms/step - loss: 1.5654
Epoch 50/50
313/313 [==============================] - 57s 182ms/step - loss: 1.5620
<tensorflow.python.keras.callbacks.History at 0x7f8d45d95d10>
To use the model with different batch sizes, we need to create a stateless copy. We can get rid of dropout since it is only used during training:
stateless_model = keras.models.Sequential([
keras.layers.GRU(128, return_sequences=True, input_shape=[None, max_id]),
keras.layers.GRU(128, return_sequences=True),
keras.layers.TimeDistributed(keras.layers.Dense(max_id,
activation="softmax"))
])
To set the weights, we first need to build the model (so the weights get created):
stateless_model.build(tf.TensorShape([None, None, max_id]))
stateless_model.set_weights(model.get_weights())
model = stateless_model
tf.random.set_seed(42)
print(complete_text("t"))
tor:
in the negver up how it thou like him;
when it
Sentiment Analysis#
tf.random.set_seed(42)
You can load the IMDB dataset easily:
(X_train, y_train), (X_test, y_test) = keras.datasets.imdb.load_data()
Downloading data from https://storage.googleapis.com/tensorflow/tf-keras-datasets/imdb.npz
17465344/17464789 [==============================] - 0s 0us/step
X_train[0][:10]
[1, 14, 22, 16, 43, 530, 973, 1622, 1385, 65]
word_index = keras.datasets.imdb.get_word_index()
id_to_word = {id_ + 3: word for word, id_ in word_index.items()}
for id_, token in enumerate(("<pad>", "<sos>", "<unk>")):
id_to_word[id_] = token
" ".join([id_to_word[id_] for id_ in X_train[0][:10]])
Downloading data from https://storage.googleapis.com/tensorflow/tf-keras-datasets/imdb_word_index.json
1646592/1641221 [==============================] - 0s 0us/step
'<sos> this film was just brilliant casting location scenery story'
import tensorflow_datasets as tfds
datasets, info = tfds.load("imdb_reviews", as_supervised=True, with_info=True)
Downloading and preparing dataset imdb_reviews/plain_text/1.0.0 (download: 80.23 MiB, generated: Unknown size, total: 80.23 MiB) to /home/aurelien_geron_kiwisoft_io/tensorflow_datasets/imdb_reviews/plain_text/1.0.0...
Shuffling and writing examples to /home/aurelien_geron_kiwisoft_io/tensorflow_datasets/imdb_reviews/plain_text/1.0.0.incompleteK5RNB1/imdb_reviews-train.tfrecord
Shuffling and writing examples to /home/aurelien_geron_kiwisoft_io/tensorflow_datasets/imdb_reviews/plain_text/1.0.0.incompleteK5RNB1/imdb_reviews-test.tfrecord
Shuffling and writing examples to /home/aurelien_geron_kiwisoft_io/tensorflow_datasets/imdb_reviews/plain_text/1.0.0.incompleteK5RNB1/imdb_reviews-unsupervised.tfrecord
Dataset imdb_reviews downloaded and prepared to /home/aurelien_geron_kiwisoft_io/tensorflow_datasets/imdb_reviews/plain_text/1.0.0. Subsequent calls will reuse this data.
datasets.keys()
dict_keys(['test', 'train', 'unsupervised'])
train_size = info.splits["train"].num_examples
test_size = info.splits["test"].num_examples
train_size, test_size
(25000, 25000)
for X_batch, y_batch in datasets["train"].batch(2).take(1):
for review, label in zip(X_batch.numpy(), y_batch.numpy()):
print("Review:", review.decode("utf-8")[:200], "...")
print("Label:", label, "= Positive" if label else "= Negative")
print()
Review: This was an absolutely terrible movie. Don't be lured in by Christopher Walken or Michael Ironside. Both are great actors, but this must simply be their worst role in history. Even their great acting ...
Label: 0 = Negative
Review: I have been known to fall asleep during films, but this is usually due to a combination of things including, really tired, being warm and comfortable on the sette and having just eaten a lot. However ...
Label: 0 = Negative
def preprocess(X_batch, y_batch):
X_batch = tf.strings.substr(X_batch, 0, 300)
X_batch = tf.strings.regex_replace(X_batch, rb"<br\s*/?>", b" ")
X_batch = tf.strings.regex_replace(X_batch, b"[^a-zA-Z']", b" ")
X_batch = tf.strings.split(X_batch)
return X_batch.to_tensor(default_value=b"<pad>"), y_batch
preprocess(X_batch, y_batch)
(<tf.Tensor: shape=(2, 53), dtype=string, numpy=
array([[b'This', b'was', b'an', b'absolutely', b'terrible', b'movie',
b"Don't", b'be', b'lured', b'in', b'by', b'Christopher',
b'Walken', b'or', b'Michael', b'Ironside', b'Both', b'are',
b'great', b'actors', b'but', b'this', b'must', b'simply', b'be',
b'their', b'worst', b'role', b'in', b'history', b'Even',
b'their', b'great', b'acting', b'could', b'not', b'redeem',
b'this', b"movie's", b'ridiculous', b'storyline', b'This',
b'movie', b'is', b'an', b'early', b'nineties', b'US',
b'propaganda', b'pi', b'<pad>', b'<pad>', b'<pad>'],
[b'I', b'have', b'been', b'known', b'to', b'fall', b'asleep',
b'during', b'films', b'but', b'this', b'is', b'usually', b'due',
b'to', b'a', b'combination', b'of', b'things', b'including',
b'really', b'tired', b'being', b'warm', b'and', b'comfortable',
b'on', b'the', b'sette', b'and', b'having', b'just', b'eaten',
b'a', b'lot', b'However', b'on', b'this', b'occasion', b'I',
b'fell', b'asleep', b'because', b'the', b'film', b'was',
b'rubbish', b'The', b'plot', b'development', b'was', b'constant',
b'Cons']], dtype=object)>,
<tf.Tensor: shape=(2,), dtype=int64, numpy=array([0, 0])>)
from collections import Counter
vocabulary = Counter()
for X_batch, y_batch in datasets["train"].batch(32).map(preprocess):
for review in X_batch:
vocabulary.update(list(review.numpy()))
vocabulary.most_common()[:3]
[(b'<pad>', 214309), (b'the', 61137), (b'a', 38564)]
len(vocabulary)
53893
vocab_size = 10000
truncated_vocabulary = [
word for word, count in vocabulary.most_common()[:vocab_size]]
word_to_id = {word: index for index, word in enumerate(truncated_vocabulary)}
for word in b"This movie was faaaaaantastic".split():
print(word_to_id.get(word) or vocab_size)
22
12
11
10000
words = tf.constant(truncated_vocabulary)
word_ids = tf.range(len(truncated_vocabulary), dtype=tf.int64)
vocab_init = tf.lookup.KeyValueTensorInitializer(words, word_ids)
num_oov_buckets = 1000
table = tf.lookup.StaticVocabularyTable(vocab_init, num_oov_buckets)
table.lookup(tf.constant([b"This movie was faaaaaantastic".split()]))
<tf.Tensor: shape=(1, 4), dtype=int64, numpy=array([[ 22, 12, 11, 10053]])>
def encode_words(X_batch, y_batch):
return table.lookup(X_batch), y_batch
train_set = datasets["train"].batch(32).map(preprocess)
train_set = train_set.map(encode_words).prefetch(1)
for X_batch, y_batch in train_set.take(1):
print(X_batch)
print(y_batch)
tf.Tensor(
[[ 22 11 28 ... 0 0 0]
[ 6 21 70 ... 0 0 0]
[4099 6881 1 ... 0 0 0]
...
[ 22 12 118 ... 331 1047 0]
[1757 4101 451 ... 0 0 0]
[3365 4392 6 ... 0 0 0]], shape=(32, 60), dtype=int64)
tf.Tensor([0 0 0 1 1 1 0 0 0 0 0 1 1 0 1 0 1 1 1 0 1 1 1 1 1 0 0 0 1 0 0 0], shape=(32,), dtype=int64)
embed_size = 128
model = keras.models.Sequential([
keras.layers.Embedding(vocab_size + num_oov_buckets, embed_size,
mask_zero=True, # not shown in the book
input_shape=[None]),
keras.layers.GRU(128, return_sequences=True),
keras.layers.GRU(128),
keras.layers.Dense(1, activation="sigmoid")
])
model.compile(loss="binary_crossentropy", optimizer="adam", metrics=["accuracy"])
history = model.fit(train_set, epochs=5)
Epoch 1/5
782/782 [==============================] - 118s 152ms/step - loss: 0.5305 - accuracy: 0.7282
Epoch 2/5
782/782 [==============================] - 113s 145ms/step - loss: 0.3459 - accuracy: 0.8554
Epoch 3/5
782/782 [==============================] - 113s 145ms/step - loss: 0.1913 - accuracy: 0.9319
Epoch 4/5
782/782 [==============================] - 114s 146ms/step - loss: 0.1341 - accuracy: 0.9535
Epoch 5/5
782/782 [==============================] - 116s 148ms/step - loss: 0.1011 - accuracy: 0.9624
Or using manual masking:
K = keras.backend
embed_size = 128
inputs = keras.layers.Input(shape=[None])
mask = keras.layers.Lambda(lambda inputs: K.not_equal(inputs, 0))(inputs)
z = keras.layers.Embedding(vocab_size + num_oov_buckets, embed_size)(inputs)
z = keras.layers.GRU(128, return_sequences=True)(z, mask=mask)
z = keras.layers.GRU(128)(z, mask=mask)
outputs = keras.layers.Dense(1, activation="sigmoid")(z)
model = keras.models.Model(inputs=[inputs], outputs=[outputs])
model.compile(loss="binary_crossentropy", optimizer="adam", metrics=["accuracy"])
history = model.fit(train_set, epochs=5)
Epoch 1/5
782/782 [==============================] - 118s 152ms/step - loss: 0.5425 - accuracy: 0.7155
Epoch 2/5
782/782 [==============================] - 112s 143ms/step - loss: 0.3479 - accuracy: 0.8558
Epoch 3/5
782/782 [==============================] - 112s 144ms/step - loss: 0.1761 - accuracy: 0.9388
Epoch 4/5
782/782 [==============================] - 115s 147ms/step - loss: 0.1281 - accuracy: 0.9531
Epoch 5/5
782/782 [==============================] - 116s 148ms/step - loss: 0.1088 - accuracy: 0.9603
Reusing Pretrained Embeddings#
tf.random.set_seed(42)
TFHUB_CACHE_DIR = os.path.join(os.curdir, "my_tfhub_cache")
os.environ["TFHUB_CACHE_DIR"] = TFHUB_CACHE_DIR
import tensorflow_hub as hub
model = keras.Sequential([
hub.KerasLayer("https://tfhub.dev/google/tf2-preview/nnlm-en-dim50/1",
dtype=tf.string, input_shape=[], output_shape=[50]),
keras.layers.Dense(128, activation="relu"),
keras.layers.Dense(1, activation="sigmoid")
])
model.compile(loss="binary_crossentropy", optimizer="adam",
metrics=["accuracy"])
for dirpath, dirnames, filenames in os.walk(TFHUB_CACHE_DIR):
for filename in filenames:
print(os.path.join(dirpath, filename))
./my_tfhub_cache/82c4aaf4250ffb09088bd48368ee7fd00e5464fe.descriptor.txt
./my_tfhub_cache/82c4aaf4250ffb09088bd48368ee7fd00e5464fe/saved_model.pb
./my_tfhub_cache/82c4aaf4250ffb09088bd48368ee7fd00e5464fe/variables/variables.data-00000-of-00001
./my_tfhub_cache/82c4aaf4250ffb09088bd48368ee7fd00e5464fe/variables/variables.index
./my_tfhub_cache/82c4aaf4250ffb09088bd48368ee7fd00e5464fe/assets/tokens.txt
import tensorflow_datasets as tfds
datasets, info = tfds.load("imdb_reviews", as_supervised=True, with_info=True)
train_size = info.splits["train"].num_examples
batch_size = 32
train_set = datasets["train"].batch(batch_size).prefetch(1)
history = model.fit(train_set, epochs=5)
Epoch 1/5
782/782 [==============================] - 128s 164ms/step - loss: 0.5460 - accuracy: 0.7267
Epoch 2/5
782/782 [==============================] - 128s 164ms/step - loss: 0.5129 - accuracy: 0.7495
Epoch 3/5
782/782 [==============================] - 129s 165ms/step - loss: 0.5082 - accuracy: 0.7530
Epoch 4/5
782/782 [==============================] - 128s 164ms/step - loss: 0.5047 - accuracy: 0.7533
Epoch 5/5
782/782 [==============================] - 128s 164ms/step - loss: 0.5015 - accuracy: 0.7560
Automatic Translation#
tf.random.set_seed(42)
vocab_size = 100
embed_size = 10
import tensorflow_addons as tfa
encoder_inputs = keras.layers.Input(shape=[None], dtype=np.int32)
decoder_inputs = keras.layers.Input(shape=[None], dtype=np.int32)
sequence_lengths = keras.layers.Input(shape=[], dtype=np.int32)
embeddings = keras.layers.Embedding(vocab_size, embed_size)
encoder_embeddings = embeddings(encoder_inputs)
decoder_embeddings = embeddings(decoder_inputs)
encoder = keras.layers.LSTM(512, return_state=True)
encoder_outputs, state_h, state_c = encoder(encoder_embeddings)
encoder_state = [state_h, state_c]
sampler = tfa.seq2seq.sampler.TrainingSampler()
decoder_cell = keras.layers.LSTMCell(512)
output_layer = keras.layers.Dense(vocab_size)
decoder = tfa.seq2seq.basic_decoder.BasicDecoder(decoder_cell, sampler,
output_layer=output_layer)
final_outputs, final_state, final_sequence_lengths = decoder(
decoder_embeddings, initial_state=encoder_state,
sequence_length=sequence_lengths)
Y_proba = tf.nn.softmax(final_outputs.rnn_output)
model = keras.models.Model(
inputs=[encoder_inputs, decoder_inputs, sequence_lengths],
outputs=[Y_proba])
model.compile(loss="sparse_categorical_crossentropy", optimizer="adam")
X = np.random.randint(100, size=10*1000).reshape(1000, 10)
Y = np.random.randint(100, size=15*1000).reshape(1000, 15)
X_decoder = np.c_[np.zeros((1000, 1)), Y[:, :-1]]
seq_lengths = np.full([1000], 15)
history = model.fit([X, X_decoder, seq_lengths], Y, epochs=2)
Epoch 1/2
32/32 [==============================] - 6s 6ms/sample - loss: 4.6053
Epoch 2/2
32/32 [==============================] - 3s 3ms/sample - loss: 4.6031
Bidirectional Recurrent Layers#
model = keras.models.Sequential([
keras.layers.GRU(10, return_sequences=True, input_shape=[None, 10]),
keras.layers.Bidirectional(keras.layers.GRU(10, return_sequences=True))
])
model.summary()
Model: "sequential_5"
_________________________________________________________________
Layer (type) Output Shape Param #
=================================================================
gru_10 (GRU) (None, None, 10) 660
_________________________________________________________________
bidirectional (Bidirectional (None, None, 20) 1320
=================================================================
Total params: 1,980
Trainable params: 1,980
Non-trainable params: 0
_________________________________________________________________
Positional Encoding#
class PositionalEncoding(keras.layers.Layer):
def __init__(self, max_steps, max_dims, dtype=tf.float32, **kwargs):
super().__init__(dtype=dtype, **kwargs)
if max_dims % 2 == 1: max_dims += 1 # max_dims must be even
p, i = np.meshgrid(np.arange(max_steps), np.arange(max_dims // 2))
pos_emb = np.empty((1, max_steps, max_dims))
pos_emb[0, :, ::2] = np.sin(p / 10000**(2 * i / max_dims)).T
pos_emb[0, :, 1::2] = np.cos(p / 10000**(2 * i / max_dims)).T
self.positional_embedding = tf.constant(pos_emb.astype(self.dtype))
def call(self, inputs):
shape = tf.shape(inputs)
return inputs + self.positional_embedding[:, :shape[-2], :shape[-1]]
max_steps = 201
max_dims = 512
pos_emb = PositionalEncoding(max_steps, max_dims)
PE = pos_emb(np.zeros((1, max_steps, max_dims), np.float32))[0].numpy()
i1, i2, crop_i = 100, 101, 150
p1, p2, p3 = 22, 60, 35
fig, (ax1, ax2) = plt.subplots(nrows=2, ncols=1, sharex=True, figsize=(9, 5))
ax1.plot([p1, p1], [-1, 1], "k--", label="$p = {}$".format(p1))
ax1.plot([p2, p2], [-1, 1], "k--", label="$p = {}$".format(p2), alpha=0.5)
ax1.plot(p3, PE[p3, i1], "bx", label="$p = {}$".format(p3))
ax1.plot(PE[:,i1], "b-", label="$i = {}$".format(i1))
ax1.plot(PE[:,i2], "r-", label="$i = {}$".format(i2))
ax1.plot([p1, p2], [PE[p1, i1], PE[p2, i1]], "bo")
ax1.plot([p1, p2], [PE[p1, i2], PE[p2, i2]], "ro")
ax1.legend(loc="center right", fontsize=14, framealpha=0.95)
ax1.set_ylabel("$P_{(p,i)}$", rotation=0, fontsize=16)
ax1.grid(True, alpha=0.3)
ax1.hlines(0, 0, max_steps - 1, color="k", linewidth=1, alpha=0.3)
ax1.axis([0, max_steps - 1, -1, 1])
ax2.imshow(PE.T[:crop_i], cmap="gray", interpolation="bilinear", aspect="auto")
ax2.hlines(i1, 0, max_steps - 1, color="b")
cheat = 2 # need to raise the red line a bit, or else it hides the blue one
ax2.hlines(i2+cheat, 0, max_steps - 1, color="r")
ax2.plot([p1, p1], [0, crop_i], "k--")
ax2.plot([p2, p2], [0, crop_i], "k--", alpha=0.5)
ax2.plot([p1, p2], [i2+cheat, i2+cheat], "ro")
ax2.plot([p1, p2], [i1, i1], "bo")
ax2.axis([0, max_steps - 1, 0, crop_i])
ax2.set_xlabel("$p$", fontsize=16)
ax2.set_ylabel("$i$", rotation=0, fontsize=16)
save_fig("positional_embedding_plot")
plt.show()
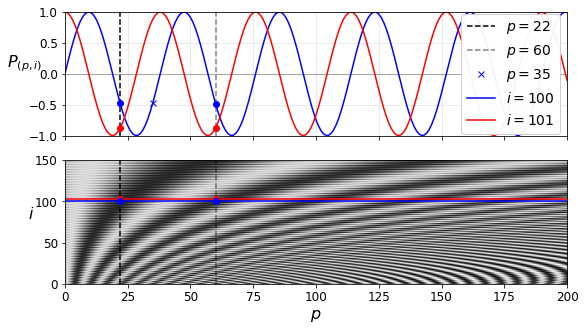
embed_size = 512; max_steps = 500; vocab_size = 10000
encoder_inputs = keras.layers.Input(shape=[None], dtype=np.int32)
decoder_inputs = keras.layers.Input(shape=[None], dtype=np.int32)
embeddings = keras.layers.Embedding(vocab_size, embed_size)
encoder_embeddings = embeddings(encoder_inputs)
decoder_embeddings = embeddings(decoder_inputs)
positional_encoding = PositionalEncoding(max_steps, max_dims=embed_size)
encoder_in = positional_encoding(encoder_embeddings)
decoder_in = positional_encoding(decoder_embeddings)
Here is a (very) simplified Transformer (the actual architecture has skip connections, layer norm, dense nets, and most importantly it uses Multi-Head Attention instead of regular Attention):
Z = encoder_in
for N in range(6):
Z = keras.layers.Attention(use_scale=True)([Z, Z])
encoder_outputs = Z
Z = decoder_in
for N in range(6):
Z = keras.layers.Attention(use_scale=True, causal=True)([Z, Z])
Z = keras.layers.Attention(use_scale=True)([Z, encoder_outputs])
outputs = keras.layers.TimeDistributed(
keras.layers.Dense(vocab_size, activation="softmax"))(Z)
Here’s a basic implementation of the MultiHeadAttention
layer. One will likely be added to keras.layers
in the near future. Note that Conv1D
layers with kernel_size=1
(and the default padding="valid"
and strides=1
) is equivalent to a TimeDistributed(Dense(...))
layer.
K = keras.backend
class MultiHeadAttention(keras.layers.Layer):
def __init__(self, n_heads, causal=False, use_scale=False, **kwargs):
self.n_heads = n_heads
self.causal = causal
self.use_scale = use_scale
super().__init__(**kwargs)
def build(self, batch_input_shape):
self.dims = batch_input_shape[0][-1]
self.q_dims, self.v_dims, self.k_dims = [self.dims // self.n_heads] * 3 # could be hyperparameters instead
self.q_linear = keras.layers.Conv1D(self.n_heads * self.q_dims, kernel_size=1, use_bias=False)
self.v_linear = keras.layers.Conv1D(self.n_heads * self.v_dims, kernel_size=1, use_bias=False)
self.k_linear = keras.layers.Conv1D(self.n_heads * self.k_dims, kernel_size=1, use_bias=False)
self.attention = keras.layers.Attention(causal=self.causal, use_scale=self.use_scale)
self.out_linear = keras.layers.Conv1D(self.dims, kernel_size=1, use_bias=False)
super().build(batch_input_shape)
def _multi_head_linear(self, inputs, linear):
shape = K.concatenate([K.shape(inputs)[:-1], [self.n_heads, -1]])
projected = K.reshape(linear(inputs), shape)
perm = K.permute_dimensions(projected, [0, 2, 1, 3])
return K.reshape(perm, [shape[0] * self.n_heads, shape[1], -1])
def call(self, inputs):
q = inputs[0]
v = inputs[1]
k = inputs[2] if len(inputs) > 2 else v
shape = K.shape(q)
q_proj = self._multi_head_linear(q, self.q_linear)
v_proj = self._multi_head_linear(v, self.v_linear)
k_proj = self._multi_head_linear(k, self.k_linear)
multi_attended = self.attention([q_proj, v_proj, k_proj])
shape_attended = K.shape(multi_attended)
reshaped_attended = K.reshape(multi_attended, [shape[0], self.n_heads, shape_attended[1], shape_attended[2]])
perm = K.permute_dimensions(reshaped_attended, [0, 2, 1, 3])
concat = K.reshape(perm, [shape[0], shape_attended[1], -1])
return self.out_linear(concat)
Q = np.random.rand(2, 50, 512)
V = np.random.rand(2, 80, 512)
multi_attn = MultiHeadAttention(8)
multi_attn([Q, V]).shape
WARNING:tensorflow:Layer multi_head_attention is casting an input tensor from dtype float64 to the layer's dtype of float32, which is new behavior in TensorFlow 2. The layer has dtype float32 because it's dtype defaults to floatx.
If you intended to run this layer in float32, you can safely ignore this warning. If in doubt, this warning is likely only an issue if you are porting a TensorFlow 1.X model to TensorFlow 2.
To change all layers to have dtype float64 by default, call `tf.keras.backend.set_floatx('float64')`. To change just this layer, pass dtype='float64' to the layer constructor. If you are the author of this layer, you can disable autocasting by passing autocast=False to the base Layer constructor.
WARNING:tensorflow:Layer multi_head_attention is casting an input tensor from dtype float64 to the layer's dtype of float32, which is new behavior in TensorFlow 2. The layer has dtype float32 because it's dtype defaults to floatx.
If you intended to run this layer in float32, you can safely ignore this warning. If in doubt, this warning is likely only an issue if you are porting a TensorFlow 1.X model to TensorFlow 2.
To change all layers to have dtype float64 by default, call `tf.keras.backend.set_floatx('float64')`. To change just this layer, pass dtype='float64' to the layer constructor. If you are the author of this layer, you can disable autocasting by passing autocast=False to the base Layer constructor.
TensorShape([2, 50, 512])
Exercise solutions#
1. to 7.#
See Appendix A.
8.#
Exercise: Embedded Reber grammars were used by Hochreiter and Schmidhuber in their paper about LSTMs. They are artificial grammars that produce strings such as “BPBTSXXVPSEPE.” Check out Jenny Orr’s nice introduction to this topic. Choose a particular embedded Reber grammar (such as the one represented on Jenny Orr’s page), then train an RNN to identify whether a string respects that grammar or not. You will first need to write a function capable of generating a training batch containing about 50% strings that respect the grammar, and 50% that don’t.
First we need to build a function that generates strings based on a grammar. The grammar will be represented as a list of possible transitions for each state. A transition specifies the string to output (or a grammar to generate it) and the next state.
default_reber_grammar = [
[("B", 1)], # (state 0) =B=>(state 1)
[("T", 2), ("P", 3)], # (state 1) =T=>(state 2) or =P=>(state 3)
[("S", 2), ("X", 4)], # (state 2) =S=>(state 2) or =X=>(state 4)
[("T", 3), ("V", 5)], # and so on...
[("X", 3), ("S", 6)],
[("P", 4), ("V", 6)],
[("E", None)]] # (state 6) =E=>(terminal state)
embedded_reber_grammar = [
[("B", 1)],
[("T", 2), ("P", 3)],
[(default_reber_grammar, 4)],
[(default_reber_grammar, 5)],
[("T", 6)],
[("P", 6)],
[("E", None)]]
def generate_string(grammar):
state = 0
output = []
while state is not None:
index = np.random.randint(len(grammar[state]))
production, state = grammar[state][index]
if isinstance(production, list):
production = generate_string(grammar=production)
output.append(production)
return "".join(output)
Let’s generate a few strings based on the default Reber grammar:
np.random.seed(42)
for _ in range(25):
print(generate_string(default_reber_grammar), end=" ")
BTXXTTVPXTVPXTTVPSE BPVPSE BTXSE BPVVE BPVVE BTSXSE BPTVPXTTTVVE BPVVE BTXSE BTXXVPSE BPTTTTTTTTVVE BTXSE BPVPSE BTXSE BPTVPSE BTXXTVPSE BPVVE BPVVE BPVVE BPTTVVE BPVVE BPVVE BTXXVVE BTXXVVE BTXXVPXVVE
Looks good. Now let’s generate a few strings based on the embedded Reber grammar:
np.random.seed(42)
for _ in range(25):
print(generate_string(embedded_reber_grammar), end=" ")
BTBPTTTVPXTVPXTTVPSETE BPBPTVPSEPE BPBPVVEPE BPBPVPXVVEPE BPBTXXTTTTVVEPE BPBPVPSEPE BPBTXXVPSEPE BPBTSSSSSSSXSEPE BTBPVVETE BPBTXXVVEPE BPBTXXVPSEPE BTBTXXVVETE BPBPVVEPE BPBPVVEPE BPBTSXSEPE BPBPVVEPE BPBPTVPSEPE BPBTXXVVEPE BTBPTVPXVVETE BTBPVVETE BTBTSSSSSSSXXVVETE BPBTSSSXXTTTTVPSEPE BTBPTTVVETE BPBTXXTVVEPE BTBTXSETE
Okay, now we need a function to generate strings that do not respect the grammar. We could generate a random string, but the task would be a bit too easy, so instead we will generate a string that respects the grammar, and we will corrupt it by changing just one character:
POSSIBLE_CHARS = "BEPSTVX"
def generate_corrupted_string(grammar, chars=POSSIBLE_CHARS):
good_string = generate_string(grammar)
index = np.random.randint(len(good_string))
good_char = good_string[index]
bad_char = np.random.choice(sorted(set(chars) - set(good_char)))
return good_string[:index] + bad_char + good_string[index + 1:]
Let’s look at a few corrupted strings:
np.random.seed(42)
for _ in range(25):
print(generate_corrupted_string(embedded_reber_grammar), end=" ")
BTBPTTTPPXTVPXTTVPSETE BPBTXEEPE BPBPTVVVEPE BPBTSSSSXSETE BPTTXSEPE BTBPVPXTTTTTTEVETE BPBTXXSVEPE BSBPTTVPSETE BPBXVVEPE BEBTXSETE BPBPVPSXPE BTBPVVVETE BPBTSXSETE BPBPTTTPTTTTTVPSEPE BTBTXXTTSTVPSETE BBBTXSETE BPBTPXSEPE BPBPVPXTTTTVPXTVPXVPXTTTVVEVE BTBXXXTVPSETE BEBTSSSSSXXVPXTVVETE BTBXTTVVETE BPBTXSTPE BTBTXXTTTVPSBTE BTBTXSETX BTBTSXSSTE
We cannot feed strings directly to an RNN, so we need to encode them somehow. One option would be to one-hot encode each character. Another option is to use embeddings. Let’s go for the second option (but since there are just a handful of characters, one-hot encoding would probably be a good option as well). For embeddings to work, we need to convert each string into a sequence of character IDs. Let’s write a function for that, using each character’s index in the string of possible characters “BEPSTVX”:
def string_to_ids(s, chars=POSSIBLE_CHARS):
return [chars.index(c) for c in s]
string_to_ids("BTTTXXVVETE")
[0, 4, 4, 4, 6, 6, 5, 5, 1, 4, 1]
We can now generate the dataset, with 50% good strings, and 50% bad strings:
def generate_dataset(size):
good_strings = [string_to_ids(generate_string(embedded_reber_grammar))
for _ in range(size // 2)]
bad_strings = [string_to_ids(generate_corrupted_string(embedded_reber_grammar))
for _ in range(size - size // 2)]
all_strings = good_strings + bad_strings
X = tf.ragged.constant(all_strings, ragged_rank=1)
y = np.array([[1.] for _ in range(len(good_strings))] +
[[0.] for _ in range(len(bad_strings))])
return X, y
np.random.seed(42)
X_train, y_train = generate_dataset(10000)
X_valid, y_valid = generate_dataset(2000)
Let’s take a look at the first training sequence:
X_train[0]
<tf.Tensor: shape=(22,), dtype=int32, numpy=
array([0, 4, 0, 2, 4, 4, 4, 5, 2, 6, 4, 5, 2, 6, 4, 4, 5, 2, 3, 1, 4, 1],
dtype=int32)>
What class does it belong to?
y_train[0]
array([1.])
Perfect! We are ready to create the RNN to identify good strings. We build a simple sequence binary classifier:
np.random.seed(42)
tf.random.set_seed(42)
embedding_size = 5
model = keras.models.Sequential([
keras.layers.InputLayer(input_shape=[None], dtype=tf.int32, ragged=True),
keras.layers.Embedding(input_dim=len(POSSIBLE_CHARS), output_dim=embedding_size),
keras.layers.GRU(30),
keras.layers.Dense(1, activation="sigmoid")
])
optimizer = keras.optimizers.SGD(learning_rate=0.02, momentum = 0.95, nesterov=True)
model.compile(loss="binary_crossentropy", optimizer=optimizer, metrics=["accuracy"])
history = model.fit(X_train, y_train, epochs=20, validation_data=(X_valid, y_valid))
Epoch 1/20
/Users/ageron/miniconda3/envs/tf2/lib/python3.7/site-packages/tensorflow_core/python/framework/indexed_slices.py:433: UserWarning: Converting sparse IndexedSlices to a dense Tensor of unknown shape. This may consume a large amount of memory.
"Converting sparse IndexedSlices to a dense Tensor of unknown shape. "
/Users/ageron/miniconda3/envs/tf2/lib/python3.7/site-packages/tensorflow_core/python/framework/indexed_slices.py:433: UserWarning: Converting sparse IndexedSlices to a dense Tensor of unknown shape. This may consume a large amount of memory.
"Converting sparse IndexedSlices to a dense Tensor of unknown shape. "
313/313 [========================================================================================================================================================================================================================================================================================================================================================================] - 5s 42us/sample - loss: 0.6847 - accuracy: 0.5138 - val_loss: 8.1518 - val_accuracy: 0.6115
Epoch 2/20
313/313 [========================================================================================================================================================================================================================================================================================================================================================================] - 3s 28us/sample - loss: 0.6524 - accuracy: 0.5571 - val_loss: 7.9259 - val_accuracy: 0.6085
Epoch 3/20
313/313 [========================================================================================================================================================================================================================================================================================================================================================================] - 3s 28us/sample - loss: 0.6686 - accuracy: 0.5783 - val_loss: 7.7483 - val_accuracy: 0.6110
Epoch 4/20
313/313 [========================================================================================================================================================================================================================================================================================================================================================================] - 3s 28us/sample - loss: 0.6201 - accuracy: 0.5969 - val_loss: 7.5567 - val_accuracy: 0.6110
Epoch 5/20
313/313 [========================================================================================================================================================================================================================================================================================================================================================================] - 3s 28us/sample - loss: 0.5705 - accuracy: 0.6428 - val_loss: 6.9117 - val_accuracy: 0.7075
Epoch 6/20
313/313 [========================================================================================================================================================================================================================================================================================================================================================================] - 3s 29us/sample - loss: 0.5660 - accuracy: 0.7008 - val_loss: 5.7277 - val_accuracy: 0.7580
Epoch 7/20
313/313 [========================================================================================================================================================================================================================================================================================================================================================================] - 3s 28us/sample - loss: 0.3997 - accuracy: 0.8336 - val_loss: 4.3641 - val_accuracy: 0.8550
Epoch 8/20
313/313 [========================================================================================================================================================================================================================================================================================================================================================================] - 3s 29us/sample - loss: 0.1771 - accuracy: 0.8958 - val_loss: 1.5009 - val_accuracy: 0.9605
Epoch 9/20
313/313 [========================================================================================================================================================================================================================================================================================================================================================================] - 3s 29us/sample - loss: 0.2710 - accuracy: 0.9566 - val_loss: 3.2648 - val_accuracy: 0.9005
Epoch 10/20
313/313 [========================================================================================================================================================================================================================================================================================================================================================================] - 3s 29us/sample - loss: 0.2574 - accuracy: 0.9620 - val_loss: 1.0385 - val_accuracy: 0.9790
Epoch 11/20
313/313 [========================================================================================================================================================================================================================================================================================================================================================================] - 3s 29us/sample - loss: 0.0356 - accuracy: 0.9845 - val_loss: 0.1081 - val_accuracy: 1.0000
Epoch 12/20
313/313 [========================================================================================================================================================================================================================================================================================================================================================================] - 4s 29us/sample - loss: 0.0029 - accuracy: 1.0000 - val_loss: 0.0261 - val_accuracy: 1.0000
Epoch 13/20
313/313 [========================================================================================================================================================================================================================================================================================================================================================================] - 3s 29us/sample - loss: 0.0019 - accuracy: 1.0000 - val_loss: 0.0144 - val_accuracy: 1.0000
Epoch 14/20
313/313 [========================================================================================================================================================================================================================================================================================================================================================================] - 3s 28us/sample - loss: 8.1710e-04 - accuracy: 1.0000 - val_loss: 0.0101 - val_accuracy: 1.0000
Epoch 15/20
313/313 [========================================================================================================================================================================================================================================================================================================================================================================] - 3s 29us/sample - loss: 5.8225e-04 - accuracy: 1.0000 - val_loss: 0.0079 - val_accuracy: 1.0000
Epoch 16/20
313/313 [========================================================================================================================================================================================================================================================================================================================================================================] - 3s 29us/sample - loss: 5.8369e-04 - accuracy: 1.0000 - val_loss: 0.0064 - val_accuracy: 1.0000
Epoch 17/20
313/313 [========================================================================================================================================================================================================================================================================================================================================================================] - 4s 30us/sample - loss: 3.8744e-04 - accuracy: 1.0000 - val_loss: 0.0054 - val_accuracy: 1.0000
Epoch 18/20
313/313 [========================================================================================================================================================================================================================================================================================================================================================================] - 4s 29us/sample - loss: 4.2988e-04 - accuracy: 1.0000 - val_loss: 0.0047 - val_accuracy: 1.0000
Epoch 19/20
313/313 [========================================================================================================================================================================================================================================================================================================================================================================] - 4s 29us/sample - loss: 2.7449e-04 - accuracy: 1.0000 - val_loss: 0.0041 - val_accuracy: 1.0000
Epoch 20/20
313/313 [========================================================================================================================================================================================================================================================================================================================================================================] - 3s 29us/sample - loss: 2.9469e-04 - accuracy: 1.0000 - val_loss: 0.0037 - val_accuracy: 1.0000
Now let’s test our RNN on two tricky strings: the first one is bad while the second one is good. They only differ by the second to last character. If the RNN gets this right, it shows that it managed to notice the pattern that the second letter should always be equal to the second to last letter. That requires a fairly long short-term memory (which is the reason why we used a GRU cell).
test_strings = ["BPBTSSSSSSSXXTTVPXVPXTTTTTVVETE",
"BPBTSSSSSSSXXTTVPXVPXTTTTTVVEPE"]
X_test = tf.ragged.constant([string_to_ids(s) for s in test_strings], ragged_rank=1)
y_proba = model.predict(X_test)
print()
print("Estimated probability that these are Reber strings:")
for index, string in enumerate(test_strings):
print("{}: {:.2f}%".format(string, 100 * y_proba[index][0]))
Estimated probability that these are Reber strings:
BPBTSSSSSSSXXTTVPXVPXTTTTTVVETE: 0.40%
BPBTSSSSSSSXXTTVPXVPXTTTTTVVEPE: 99.96%
Ta-da! It worked fine. The RNN found the correct answers with very high confidence. :)
9.#
Exercise: Train an Encoder–Decoder model that can convert a date string from one format to another (e.g., from “April 22, 2019” to “2019-04-22”).
Let’s start by creating the dataset. We will use random days between 1000-01-01 and 9999-12-31:
from datetime import date
# cannot use strftime()'s %B format since it depends on the locale
MONTHS = ["January", "February", "March", "April", "May", "June",
"July", "August", "September", "October", "November", "December"]
def random_dates(n_dates):
min_date = date(1000, 1, 1).toordinal()
max_date = date(9999, 12, 31).toordinal()
ordinals = np.random.randint(max_date - min_date, size=n_dates) + min_date
dates = [date.fromordinal(ordinal) for ordinal in ordinals]
x = [MONTHS[dt.month - 1] + " " + dt.strftime("%d, %Y") for dt in dates]
y = [dt.isoformat() for dt in dates]
return x, y
Here are a few random dates, displayed in both the input format and the target format:
np.random.seed(42)
n_dates = 3
x_example, y_example = random_dates(n_dates)
print("{:25s}{:25s}".format("Input", "Target"))
print("-" * 50)
for idx in range(n_dates):
print("{:25s}{:25s}".format(x_example[idx], y_example[idx]))
Input Target
--------------------------------------------------
September 20, 7075 7075-09-20
May 15, 8579 8579-05-15
January 11, 7103 7103-01-11
Let’s get the list of all possible characters in the inputs:
INPUT_CHARS = "".join(sorted(set("".join(MONTHS) + "0123456789, ")))
INPUT_CHARS
' ,0123456789ADFJMNOSabceghilmnoprstuvy'
And here’s the list of possible characters in the outputs:
OUTPUT_CHARS = "0123456789-"
Let’s write a function to convert a string to a list of character IDs, as we did in the previous exercise:
def date_str_to_ids(date_str, chars=INPUT_CHARS):
return [chars.index(c) for c in date_str]
date_str_to_ids(x_example[0], INPUT_CHARS)
[7, 11, 19, 22, 11, 16, 9, 11, 20, 38, 28, 26, 37, 38, 33, 26, 33, 31]
date_str_to_ids(y_example[0], OUTPUT_CHARS)
[7, 0, 7, 5, 10, 0, 9, 10, 2, 0]
def prepare_date_strs(date_strs, chars=INPUT_CHARS):
X_ids = [date_str_to_ids(dt, chars) for dt in date_strs]
X = tf.ragged.constant(X_ids, ragged_rank=1)
return (X + 1).to_tensor() # using 0 as the padding token ID
def create_dataset(n_dates):
x, y = random_dates(n_dates)
return prepare_date_strs(x, INPUT_CHARS), prepare_date_strs(y, OUTPUT_CHARS)
np.random.seed(42)
X_train, Y_train = create_dataset(10000)
X_valid, Y_valid = create_dataset(2000)
X_test, Y_test = create_dataset(2000)
Y_train[0]
<tf.Tensor: shape=(10,), dtype=int32, numpy=array([ 8, 1, 8, 6, 11, 1, 10, 11, 3, 1], dtype=int32)>
First version: a very basic seq2seq model#
Let’s first try the simplest possible model: we feed in the input sequence, which first goes through the encoder (an embedding layer followed by a single LSTM layer), which outputs a vector, then it goes through a decoder (a single LSTM layer, followed by a dense output layer), which outputs a sequence of vectors, each representing the estimated probabilities for all possible output character.
Since the decoder expects a sequence as input, we repeat the vector (which is output by the decoder) as many times as the longest possible output sequence.
embedding_size = 32
max_output_length = Y_train.shape[1]
np.random.seed(42)
tf.random.set_seed(42)
encoder = keras.models.Sequential([
keras.layers.Embedding(input_dim=len(INPUT_CHARS) + 1,
output_dim=embedding_size,
input_shape=[None]),
keras.layers.LSTM(128)
])
decoder = keras.models.Sequential([
keras.layers.LSTM(128, return_sequences=True),
keras.layers.Dense(len(OUTPUT_CHARS) + 1, activation="softmax")
])
model = keras.models.Sequential([
encoder,
keras.layers.RepeatVector(max_output_length),
decoder
])
optimizer = keras.optimizers.Nadam()
model.compile(loss="sparse_categorical_crossentropy", optimizer=optimizer,
metrics=["accuracy"])
history = model.fit(X_train, Y_train, epochs=20,
validation_data=(X_valid, Y_valid))
Epoch 1/20
313/313 [==============================] - 6s 18ms/step - loss: 1.8111 - accuracy: 0.3533 - val_loss: 1.3581 - val_accuracy: 0.4965
Epoch 2/20
313/313 [==============================] - 5s 15ms/step - loss: 1.3518 - accuracy: 0.5103 - val_loss: 1.1915 - val_accuracy: 0.5694
Epoch 3/20
313/313 [==============================] - 5s 15ms/step - loss: 1.1706 - accuracy: 0.5908 - val_loss: 0.9983 - val_accuracy: 0.6398
Epoch 4/20
313/313 [==============================] - 5s 15ms/step - loss: 0.9158 - accuracy: 0.6686 - val_loss: 0.8012 - val_accuracy: 0.6987
Epoch 5/20
313/313 [==============================] - 5s 15ms/step - loss: 0.7058 - accuracy: 0.7308 - val_loss: 0.6224 - val_accuracy: 0.7599
Epoch 6/20
313/313 [==============================] - 5s 15ms/step - loss: 0.7756 - accuracy: 0.7203 - val_loss: 0.6541 - val_accuracy: 0.7599
Epoch 7/20
313/313 [==============================] - 5s 16ms/step - loss: 0.5379 - accuracy: 0.8034 - val_loss: 0.4174 - val_accuracy: 0.8440
Epoch 8/20
313/313 [==============================] - 5s 15ms/step - loss: 0.4867 - accuracy: 0.8262 - val_loss: 0.4188 - val_accuracy: 0.8480
Epoch 9/20
313/313 [==============================] - 5s 15ms/step - loss: 0.2979 - accuracy: 0.8951 - val_loss: 0.2549 - val_accuracy: 0.9126
Epoch 10/20
313/313 [==============================] - 5s 14ms/step - loss: 0.1785 - accuracy: 0.9479 - val_loss: 0.1461 - val_accuracy: 0.9594
Epoch 11/20
313/313 [==============================] - 5s 15ms/step - loss: 0.1830 - accuracy: 0.9557 - val_loss: 0.1644 - val_accuracy: 0.9550
Epoch 12/20
313/313 [==============================] - 5s 15ms/step - loss: 0.0775 - accuracy: 0.9857 - val_loss: 0.0595 - val_accuracy: 0.9901
Epoch 13/20
313/313 [==============================] - 5s 15ms/step - loss: 0.0400 - accuracy: 0.9953 - val_loss: 0.0342 - val_accuracy: 0.9957
Epoch 14/20
313/313 [==============================] - 5s 15ms/step - loss: 0.0248 - accuracy: 0.9979 - val_loss: 0.0231 - val_accuracy: 0.9983
Epoch 15/20
313/313 [==============================] - 5s 15ms/step - loss: 0.0161 - accuracy: 0.9991 - val_loss: 0.0149 - val_accuracy: 0.9995
Epoch 16/20
313/313 [==============================] - 5s 15ms/step - loss: 0.0108 - accuracy: 0.9997 - val_loss: 0.0106 - val_accuracy: 0.9996
Epoch 17/20
313/313 [==============================] - 5s 15ms/step - loss: 0.0074 - accuracy: 0.9999 - val_loss: 0.0077 - val_accuracy: 0.9999
Epoch 18/20
313/313 [==============================] - 5s 15ms/step - loss: 0.0053 - accuracy: 1.0000 - val_loss: 0.0054 - val_accuracy: 0.9999
Epoch 19/20
313/313 [==============================] - 5s 15ms/step - loss: 0.0039 - accuracy: 1.0000 - val_loss: 0.0041 - val_accuracy: 1.0000
Epoch 20/20
313/313 [==============================] - 5s 15ms/step - loss: 0.0029 - accuracy: 1.0000 - val_loss: 0.0032 - val_accuracy: 1.0000
Looks great, we reach 100% validation accuracy! Let’s use the model to make some predictions. We will need to be able to convert a sequence of character IDs to a readable string:
def ids_to_date_strs(ids, chars=OUTPUT_CHARS):
return ["".join([("?" + chars)[index] for index in sequence])
for sequence in ids]
Now we can use the model to convert some dates
X_new = prepare_date_strs(["September 17, 2009", "July 14, 1789"])
#ids = model.predict_classes(X_new)
ids = np.argmax(model.predict(X_new), axis=-1)
for date_str in ids_to_date_strs(ids):
print(date_str)
2009-09-17
1789-07-14
Perfect! :)
However, since the model was only trained on input strings of length 18 (which is the length of the longest date), it does not perform well if we try to use it to make predictions on shorter sequences:
X_new = prepare_date_strs(["May 02, 2020", "July 14, 1789"])
#ids = model.predict_classes(X_new)
ids = np.argmax(model.predict(X_new), axis=-1)
for date_str in ids_to_date_strs(ids):
print(date_str)
2020-01-02
1789-02-14
Oops! We need to ensure that we always pass sequences of the same length as during training, using padding if necessary. Let’s write a little helper function for that:
max_input_length = X_train.shape[1]
def prepare_date_strs_padded(date_strs):
X = prepare_date_strs(date_strs)
if X.shape[1] < max_input_length:
X = tf.pad(X, [[0, 0], [0, max_input_length - X.shape[1]]])
return X
def convert_date_strs(date_strs):
X = prepare_date_strs_padded(date_strs)
#ids = model.predict_classes(X)
ids = np.argmax(model.predict(X), axis=-1)
return ids_to_date_strs(ids)
convert_date_strs(["May 02, 2020", "July 14, 1789"])
['2020-05-02', '1789-07-14']
Cool! Granted, there are certainly much easier ways to write a date conversion tool (e.g., using regular expressions or even basic string manipulation), but you have to admit that using neural networks is way cooler. ;-)
However, real-life sequence-to-sequence problems will usually be harder, so for the sake of completeness, let’s build a more powerful model.
Second version: feeding the shifted targets to the decoder (teacher forcing)#
Instead of feeding the decoder a simple repetition of the encoder’s output vector, we can feed it the target sequence, shifted by one time step to the right. This way, at each time step the decoder will know what the previous target character was. This should help is tackle more complex sequence-to-sequence problems.
Since the first output character of each target sequence has no previous character, we will need a new token to represent the start-of-sequence (sos).
During inference, we won’t know the target, so what will we feed the decoder? We can just predict one character at a time, starting with an sos token, then feeding the decoder all the characters that were predicted so far (we will look at this in more details later in this notebook).
But if the decoder’s LSTM expects to get the previous target as input at each step, how shall we pass it it the vector output by the encoder? Well, one option is to ignore the output vector, and instead use the encoder’s LSTM state as the initial state of the decoder’s LSTM (which requires that encoder’s LSTM must have the same number of units as the decoder’s LSTM).
Now let’s create the decoder’s inputs (for training, validation and testing). The sos token will be represented using the last possible output character’s ID + 1.
sos_id = len(OUTPUT_CHARS) + 1
def shifted_output_sequences(Y):
sos_tokens = tf.fill(dims=(len(Y), 1), value=sos_id)
return tf.concat([sos_tokens, Y[:, :-1]], axis=1)
X_train_decoder = shifted_output_sequences(Y_train)
X_valid_decoder = shifted_output_sequences(Y_valid)
X_test_decoder = shifted_output_sequences(Y_test)
Let’s take a look at the decoder’s training inputs:
X_train_decoder
<tf.Tensor: shape=(10000, 10), dtype=int32, numpy=
array([[12, 8, 1, ..., 10, 11, 3],
[12, 9, 6, ..., 6, 11, 2],
[12, 8, 2, ..., 2, 11, 2],
...,
[12, 10, 8, ..., 2, 11, 4],
[12, 2, 2, ..., 3, 11, 3],
[12, 8, 9, ..., 8, 11, 3]], dtype=int32)>
Now let’s build the model. It’s not a simple sequential model anymore, so let’s use the functional API:
encoder_embedding_size = 32
decoder_embedding_size = 32
lstm_units = 128
np.random.seed(42)
tf.random.set_seed(42)
encoder_input = keras.layers.Input(shape=[None], dtype=tf.int32)
encoder_embedding = keras.layers.Embedding(
input_dim=len(INPUT_CHARS) + 1,
output_dim=encoder_embedding_size)(encoder_input)
_, encoder_state_h, encoder_state_c = keras.layers.LSTM(
lstm_units, return_state=True)(encoder_embedding)
encoder_state = [encoder_state_h, encoder_state_c]
decoder_input = keras.layers.Input(shape=[None], dtype=tf.int32)
decoder_embedding = keras.layers.Embedding(
input_dim=len(OUTPUT_CHARS) + 2,
output_dim=decoder_embedding_size)(decoder_input)
decoder_lstm_output = keras.layers.LSTM(lstm_units, return_sequences=True)(
decoder_embedding, initial_state=encoder_state)
decoder_output = keras.layers.Dense(len(OUTPUT_CHARS) + 1,
activation="softmax")(decoder_lstm_output)
model = keras.models.Model(inputs=[encoder_input, decoder_input],
outputs=[decoder_output])
optimizer = keras.optimizers.Nadam()
model.compile(loss="sparse_categorical_crossentropy", optimizer=optimizer,
metrics=["accuracy"])
history = model.fit([X_train, X_train_decoder], Y_train, epochs=10,
validation_data=([X_valid, X_valid_decoder], Y_valid))
Epoch 1/10
313/313 [==============================] - 5s 17ms/step - loss: 1.6898 - accuracy: 0.3714 - val_loss: 1.4141 - val_accuracy: 0.4603
Epoch 2/10
313/313 [==============================] - 5s 15ms/step - loss: 1.2118 - accuracy: 0.5541 - val_loss: 0.9360 - val_accuracy: 0.6653
Epoch 3/10
313/313 [==============================] - 5s 15ms/step - loss: 0.6399 - accuracy: 0.7766 - val_loss: 0.4054 - val_accuracy: 0.8631
Epoch 4/10
313/313 [==============================] - 5s 15ms/step - loss: 0.2207 - accuracy: 0.9463 - val_loss: 0.1069 - val_accuracy: 0.9869
Epoch 5/10
313/313 [==============================] - 5s 15ms/step - loss: 0.0805 - accuracy: 0.9910 - val_loss: 0.0445 - val_accuracy: 0.9976
Epoch 6/10
313/313 [==============================] - 5s 15ms/step - loss: 0.0297 - accuracy: 0.9993 - val_loss: 0.0237 - val_accuracy: 0.9992
Epoch 7/10
313/313 [==============================] - 5s 15ms/step - loss: 0.0743 - accuracy: 0.9857 - val_loss: 0.0702 - val_accuracy: 0.9889
Epoch 8/10
313/313 [==============================] - 5s 15ms/step - loss: 0.0187 - accuracy: 0.9995 - val_loss: 0.0112 - val_accuracy: 0.9999
Epoch 9/10
313/313 [==============================] - 5s 15ms/step - loss: 0.0084 - accuracy: 1.0000 - val_loss: 0.0072 - val_accuracy: 1.0000
Epoch 10/10
313/313 [==============================] - 5s 15ms/step - loss: 0.0057 - accuracy: 1.0000 - val_loss: 0.0053 - val_accuracy: 1.0000
This model also reaches 100% validation accuracy, but it does so even faster.
Let’s once again use the model to make some predictions. This time we need to predict characters one by one.
sos_id = len(OUTPUT_CHARS) + 1
def predict_date_strs(date_strs):
X = prepare_date_strs_padded(date_strs)
Y_pred = tf.fill(dims=(len(X), 1), value=sos_id)
for index in range(max_output_length):
pad_size = max_output_length - Y_pred.shape[1]
X_decoder = tf.pad(Y_pred, [[0, 0], [0, pad_size]])
Y_probas_next = model.predict([X, X_decoder])[:, index:index+1]
Y_pred_next = tf.argmax(Y_probas_next, axis=-1, output_type=tf.int32)
Y_pred = tf.concat([Y_pred, Y_pred_next], axis=1)
return ids_to_date_strs(Y_pred[:, 1:])
predict_date_strs(["July 14, 1789", "May 01, 2020"])
['1789-07-14', '2020-05-01']
Works fine! :)
Third version: using TF-Addons’s seq2seq implementation#
Let’s build exactly the same model, but using TF-Addon’s seq2seq API. The implementation below is almost very similar to the TFA example higher in this notebook, except without the model input to specify the output sequence length, for simplicity (but you can easily add it back in if you need it for your projects, when the output sequences have very different lengths).
import tensorflow_addons as tfa
np.random.seed(42)
tf.random.set_seed(42)
encoder_embedding_size = 32
decoder_embedding_size = 32
units = 128
encoder_inputs = keras.layers.Input(shape=[None], dtype=np.int32)
decoder_inputs = keras.layers.Input(shape=[None], dtype=np.int32)
sequence_lengths = keras.layers.Input(shape=[], dtype=np.int32)
encoder_embeddings = keras.layers.Embedding(
len(INPUT_CHARS) + 1, encoder_embedding_size)(encoder_inputs)
decoder_embedding_layer = keras.layers.Embedding(
len(OUTPUT_CHARS) + 2, decoder_embedding_size)
decoder_embeddings = decoder_embedding_layer(decoder_inputs)
encoder = keras.layers.LSTM(units, return_state=True)
encoder_outputs, state_h, state_c = encoder(encoder_embeddings)
encoder_state = [state_h, state_c]
sampler = tfa.seq2seq.sampler.TrainingSampler()
decoder_cell = keras.layers.LSTMCell(units)
output_layer = keras.layers.Dense(len(OUTPUT_CHARS) + 1)
decoder = tfa.seq2seq.basic_decoder.BasicDecoder(decoder_cell,
sampler,
output_layer=output_layer)
final_outputs, final_state, final_sequence_lengths = decoder(
decoder_embeddings,
initial_state=encoder_state)
Y_proba = keras.layers.Activation("softmax")(final_outputs.rnn_output)
model = keras.models.Model(inputs=[encoder_inputs, decoder_inputs],
outputs=[Y_proba])
optimizer = keras.optimizers.Nadam()
model.compile(loss="sparse_categorical_crossentropy", optimizer=optimizer,
metrics=["accuracy"])
history = model.fit([X_train, X_train_decoder], Y_train, epochs=15,
validation_data=([X_valid, X_valid_decoder], Y_valid))
Epoch 1/15
313/313 [==============================] - 5s 17ms/step - loss: 1.6757 - accuracy: 0.3683 - val_loss: 1.4602 - val_accuracy: 0.4214
Epoch 2/15
313/313 [==============================] - 5s 15ms/step - loss: 1.3873 - accuracy: 0.4566 - val_loss: 1.2904 - val_accuracy: 0.4957
Epoch 3/15
313/313 [==============================] - 5s 15ms/step - loss: 1.0471 - accuracy: 0.6109 - val_loss: 0.7737 - val_accuracy: 0.7276
Epoch 4/15
313/313 [==============================] - 5s 15ms/step - loss: 0.5056 - accuracy: 0.8296 - val_loss: 0.2695 - val_accuracy: 0.9305
Epoch 5/15
313/313 [==============================] - 5s 15ms/step - loss: 0.1677 - accuracy: 0.9657 - val_loss: 0.0870 - val_accuracy: 0.9912
Epoch 6/15
313/313 [==============================] - 5s 15ms/step - loss: 0.1007 - accuracy: 0.9850 - val_loss: 0.0492 - val_accuracy: 0.9975
Epoch 7/15
313/313 [==============================] - 5s 15ms/step - loss: 0.0308 - accuracy: 0.9993 - val_loss: 0.0228 - val_accuracy: 0.9996
Epoch 8/15
313/313 [==============================] - 5s 15ms/step - loss: 0.0168 - accuracy: 0.9999 - val_loss: 0.0144 - val_accuracy: 0.9999
Epoch 9/15
313/313 [==============================] - 5s 15ms/step - loss: 0.0107 - accuracy: 1.0000 - val_loss: 0.0095 - val_accuracy: 0.9999
Epoch 10/15
313/313 [==============================] - 5s 15ms/step - loss: 0.0074 - accuracy: 1.0000 - val_loss: 0.0066 - val_accuracy: 0.9999
Epoch 11/15
313/313 [==============================] - 5s 15ms/step - loss: 0.0053 - accuracy: 1.0000 - val_loss: 0.0051 - val_accuracy: 0.9999
Epoch 12/15
313/313 [==============================] - 5s 15ms/step - loss: 0.0039 - accuracy: 1.0000 - val_loss: 0.0037 - val_accuracy: 1.0000
Epoch 13/15
313/313 [==============================] - 5s 15ms/step - loss: 0.0029 - accuracy: 1.0000 - val_loss: 0.0030 - val_accuracy: 1.0000
Epoch 14/15
313/313 [==============================] - 5s 15ms/step - loss: 0.0023 - accuracy: 1.0000 - val_loss: 0.0022 - val_accuracy: 1.0000
Epoch 15/15
313/313 [==============================] - 5s 15ms/step - loss: 0.0018 - accuracy: 1.0000 - val_loss: 0.0018 - val_accuracy: 1.0000
And once again, 100% validation accuracy! To use the model, we can just reuse the predict_date_strs()
function:
predict_date_strs(["July 14, 1789", "May 01, 2020"])
['1789-07-14', '2020-05-01']
However, there’s a much more efficient way to perform inference. Until now, during inference, we’ve run the model once for each new character. Instead, we can create a new decoder, based on the previously trained layers, but using a GreedyEmbeddingSampler
instead of a TrainingSampler
.
At each time step, the GreedyEmbeddingSampler
will compute the argmax of the decoder’s outputs, and run the resulting token IDs through the decoder’s embedding layer. Then it will feed the resulting embeddings to the decoder’s LSTM cell at the next time step. This way, we only need to run the decoder once to get the full prediction.
inference_sampler = tfa.seq2seq.sampler.GreedyEmbeddingSampler(
embedding_fn=decoder_embedding_layer)
inference_decoder = tfa.seq2seq.basic_decoder.BasicDecoder(
decoder_cell, inference_sampler, output_layer=output_layer,
maximum_iterations=max_output_length)
batch_size = tf.shape(encoder_inputs)[:1]
start_tokens = tf.fill(dims=batch_size, value=sos_id)
final_outputs, final_state, final_sequence_lengths = inference_decoder(
start_tokens,
initial_state=encoder_state,
start_tokens=start_tokens,
end_token=0)
inference_model = keras.models.Model(inputs=[encoder_inputs],
outputs=[final_outputs.sample_id])
A few notes:
The
GreedyEmbeddingSampler
needs thestart_tokens
(a vector containing the start-of-sequence ID for each decoder sequence), and theend_token
(the decoder will stop decoding a sequence once the model outputs this token).We must set
maximum_iterations
when creating theBasicDecoder
, or else it may run into an infinite loop (if the model never outputs the end token for at least one of the sequences). This would force you would to restart the Jupyter kernel.The decoder inputs are not needed anymore, since all the decoder inputs are generated dynamically based on the outputs from the previous time step.
The model’s outputs are
final_outputs.sample_id
instead of the softmax offinal_outputs.rnn_outputs
. This allows us to directly get the argmax of the model’s outputs. If you prefer to have access to the logits, you can replacefinal_outputs.sample_id
withfinal_outputs.rnn_outputs
.
Now we can write a simple function that uses the model to perform the date format conversion:
def fast_predict_date_strs(date_strs):
X = prepare_date_strs_padded(date_strs)
Y_pred = inference_model.predict(X)
return ids_to_date_strs(Y_pred)
fast_predict_date_strs(["July 14, 1789", "May 01, 2020"])
['1789-07-14', '2020-05-01']
Let’s check that it really is faster:
%timeit predict_date_strs(["July 14, 1789", "May 01, 2020"])
199 ms ± 3.94 ms per loop (mean ± std. dev. of 7 runs, 1 loop each)
%timeit fast_predict_date_strs(["July 14, 1789", "May 01, 2020"])
18.3 ms ± 366 µs per loop (mean ± std. dev. of 7 runs, 10 loops each)
That’s more than a 10x speedup! And it would be even more if we were handling longer sequences.
Fourth version: using TF-Addons’s seq2seq implementation with a scheduled sampler#
Warning: due to a TF bug, this version only works using TensorFlow 2.2 or above.
When we trained the previous model, at each time step t we gave the model the target token for time step t - 1. However, at inference time, the model did not get the previous target at each time step. Instead, it got the previous prediction. So there is a discrepancy between training and inference, which may lead to disappointing performance. To alleviate this, we can gradually replace the targets with the predictions, during training. For this, we just need to replace the TrainingSampler
with a ScheduledEmbeddingTrainingSampler
, and use a Keras callback to gradually increase the sampling_probability
(i.e., the probability that the decoder will use the prediction from the previous time step rather than the target for the previous time step).
import tensorflow_addons as tfa
np.random.seed(42)
tf.random.set_seed(42)
n_epochs = 20
encoder_embedding_size = 32
decoder_embedding_size = 32
units = 128
encoder_inputs = keras.layers.Input(shape=[None], dtype=np.int32)
decoder_inputs = keras.layers.Input(shape=[None], dtype=np.int32)
sequence_lengths = keras.layers.Input(shape=[], dtype=np.int32)
encoder_embeddings = keras.layers.Embedding(
len(INPUT_CHARS) + 1, encoder_embedding_size)(encoder_inputs)
decoder_embedding_layer = keras.layers.Embedding(
len(OUTPUT_CHARS) + 2, decoder_embedding_size)
decoder_embeddings = decoder_embedding_layer(decoder_inputs)
encoder = keras.layers.LSTM(units, return_state=True)
encoder_outputs, state_h, state_c = encoder(encoder_embeddings)
encoder_state = [state_h, state_c]
sampler = tfa.seq2seq.sampler.ScheduledEmbeddingTrainingSampler(
sampling_probability=0.,
embedding_fn=decoder_embedding_layer)
# we must set the sampling_probability after creating the sampler
# (see https://github.com/tensorflow/addons/pull/1714)
sampler.sampling_probability = tf.Variable(0.)
decoder_cell = keras.layers.LSTMCell(units)
output_layer = keras.layers.Dense(len(OUTPUT_CHARS) + 1)
decoder = tfa.seq2seq.basic_decoder.BasicDecoder(decoder_cell,
sampler,
output_layer=output_layer)
final_outputs, final_state, final_sequence_lengths = decoder(
decoder_embeddings,
initial_state=encoder_state)
Y_proba = keras.layers.Activation("softmax")(final_outputs.rnn_output)
model = keras.models.Model(inputs=[encoder_inputs, decoder_inputs],
outputs=[Y_proba])
optimizer = keras.optimizers.Nadam()
model.compile(loss="sparse_categorical_crossentropy", optimizer=optimizer,
metrics=["accuracy"])
def update_sampling_probability(epoch, logs):
proba = min(1.0, epoch / (n_epochs - 10))
sampler.sampling_probability.assign(proba)
sampling_probability_cb = keras.callbacks.LambdaCallback(
on_epoch_begin=update_sampling_probability)
history = model.fit([X_train, X_train_decoder], Y_train, epochs=n_epochs,
validation_data=([X_valid, X_valid_decoder], Y_valid),
callbacks=[sampling_probability_cb])
Epoch 1/20
/Users/ageron/miniconda3/envs/tf2/lib/python3.7/site-packages/tensorflow/python/framework/indexed_slices.py:434: UserWarning: Converting sparse IndexedSlices to a dense Tensor of unknown shape. This may consume a large amount of memory.
"Converting sparse IndexedSlices to a dense Tensor of unknown shape. "
313/313 [==============================] - 6s 19ms/step - loss: 1.6759 - accuracy: 0.3681 - val_loss: 1.4611 - val_accuracy: 0.4198
Epoch 2/20
313/313 [==============================] - 5s 17ms/step - loss: 1.3872 - accuracy: 0.4583 - val_loss: 1.2827 - val_accuracy: 0.5021
Epoch 3/20
313/313 [==============================] - 5s 17ms/step - loss: 1.0425 - accuracy: 0.6152 - val_loss: 0.8165 - val_accuracy: 0.7000
Epoch 4/20
313/313 [==============================] - 5s 17ms/step - loss: 0.6353 - accuracy: 0.7673 - val_loss: 0.4365 - val_accuracy: 0.8464
Epoch 5/20
313/313 [==============================] - 5s 17ms/step - loss: 0.3764 - accuracy: 0.8765 - val_loss: 0.2795 - val_accuracy: 0.9166
Epoch 6/20
313/313 [==============================] - 5s 17ms/step - loss: 0.2506 - accuracy: 0.9269 - val_loss: 0.1805 - val_accuracy: 0.9489
Epoch 7/20
313/313 [==============================] - 5s 17ms/step - loss: 0.1427 - accuracy: 0.9625 - val_loss: 0.1115 - val_accuracy: 0.9718
Epoch 8/20
313/313 [==============================] - 5s 17ms/step - loss: 0.0853 - accuracy: 0.9804 - val_loss: 0.0785 - val_accuracy: 0.9809
Epoch 9/20
313/313 [==============================] - 5s 17ms/step - loss: 0.1010 - accuracy: 0.9797 - val_loss: 0.1198 - val_accuracy: 0.9746
Epoch 10/20
313/313 [==============================] - 5s 17ms/step - loss: 0.0447 - accuracy: 0.9917 - val_loss: 0.0306 - val_accuracy: 0.9949
Epoch 11/20
313/313 [==============================] - 5s 16ms/step - loss: 0.0241 - accuracy: 0.9961 - val_loss: 0.0205 - val_accuracy: 0.9968
Epoch 12/20
313/313 [==============================] - 5s 17ms/step - loss: 0.0705 - accuracy: 0.9861 - val_loss: 0.0823 - val_accuracy: 0.9860
Epoch 13/20
313/313 [==============================] - 5s 16ms/step - loss: 0.0182 - accuracy: 0.9977 - val_loss: 0.0117 - val_accuracy: 0.9980
Epoch 14/20
313/313 [==============================] - 5s 16ms/step - loss: 0.0088 - accuracy: 0.9990 - val_loss: 0.0085 - val_accuracy: 0.9990
Epoch 15/20
313/313 [==============================] - 5s 16ms/step - loss: 0.0059 - accuracy: 0.9994 - val_loss: 0.0061 - val_accuracy: 0.9993
Epoch 16/20
313/313 [==============================] - 5s 16ms/step - loss: 0.0045 - accuracy: 0.9996 - val_loss: 0.0048 - val_accuracy: 0.9996
Epoch 17/20
313/313 [==============================] - 5s 16ms/step - loss: 0.0038 - accuracy: 0.9997 - val_loss: 0.0039 - val_accuracy: 0.9995
Epoch 18/20
313/313 [==============================] - 5s 16ms/step - loss: 0.0029 - accuracy: 0.9997 - val_loss: 0.0024 - val_accuracy: 0.9999
Epoch 19/20
313/313 [==============================] - 5s 16ms/step - loss: 0.0020 - accuracy: 0.9999 - val_loss: 0.0031 - val_accuracy: 0.9992
Epoch 20/20
313/313 [==============================] - 5s 16ms/step - loss: 0.0018 - accuracy: 0.9999 - val_loss: 0.0022 - val_accuracy: 0.9999
Not quite 100% validation accuracy, but close enough!
For inference, we could do the exact same thing as earlier, using a GreedyEmbeddingSampler
. However, just for the sake of completeness, let’s use a SampleEmbeddingSampler
instead. It’s almost the same thing, except that instead of using the argmax of the model’s output to find the token ID, it treats the outputs as logits and uses them to sample a token ID randomly. This can be useful when you want to generate text. The softmax_temperature
argument serves the
same purpose as when we generated Shakespeare-like text (the higher this argument, the more random the generated text will be).
softmax_temperature = tf.Variable(1.)
inference_sampler = tfa.seq2seq.sampler.SampleEmbeddingSampler(
embedding_fn=decoder_embedding_layer,
softmax_temperature=softmax_temperature)
inference_decoder = tfa.seq2seq.basic_decoder.BasicDecoder(
decoder_cell, inference_sampler, output_layer=output_layer,
maximum_iterations=max_output_length)
batch_size = tf.shape(encoder_inputs)[:1]
start_tokens = tf.fill(dims=batch_size, value=sos_id)
final_outputs, final_state, final_sequence_lengths = inference_decoder(
start_tokens,
initial_state=encoder_state,
start_tokens=start_tokens,
end_token=0)
inference_model = keras.models.Model(inputs=[encoder_inputs],
outputs=[final_outputs.sample_id])
def creative_predict_date_strs(date_strs, temperature=1.0):
softmax_temperature.assign(temperature)
X = prepare_date_strs_padded(date_strs)
Y_pred = inference_model.predict(X)
return ids_to_date_strs(Y_pred)
tf.random.set_seed(42)
creative_predict_date_strs(["July 14, 1789", "May 01, 2020"])
['1789-07-14', '2020-05-01']
Dates look good at room temperature. Now let’s heat things up a bit:
tf.random.set_seed(42)
creative_predict_date_strs(["July 14, 1789", "May 01, 2020"],
temperature=5.)
['2289607-12', '9272-03-01']
Oops, the dates are overcooked, now. Let’s call them “creative” dates.
Fifth version: using TFA seq2seq, the Keras subclassing API and attention mechanisms#
The sequences in this problem are pretty short, but if we wanted to tackle longer sequences, we would probably have to use attention mechanisms. While it’s possible to code our own implementation, it’s simpler and more efficient to use TF-Addons’s implementation instead. Let’s do that now, this time using Keras’ subclassing API.
Warning: due to a TensorFlow bug (see this issue for details), the get_initial_state()
method fails in eager mode, so for now we have to use the subclassing API, as Keras automatically calls tf.function()
on the call()
method (so it runs in graph mode).
In this implementation, we’ve reverted back to using the TrainingSampler
, for simplicity (but you can easily tweak it to use a ScheduledEmbeddingTrainingSampler
instead). We also use a GreedyEmbeddingSampler
during inference, so this class is pretty easy to use:
class DateTranslation(keras.models.Model):
def __init__(self, units=128, encoder_embedding_size=32,
decoder_embedding_size=32, **kwargs):
super().__init__(**kwargs)
self.encoder_embedding = keras.layers.Embedding(
input_dim=len(INPUT_CHARS) + 1,
output_dim=encoder_embedding_size)
self.encoder = keras.layers.LSTM(units,
return_sequences=True,
return_state=True)
self.decoder_embedding = keras.layers.Embedding(
input_dim=len(OUTPUT_CHARS) + 2,
output_dim=decoder_embedding_size)
self.attention = tfa.seq2seq.LuongAttention(units)
decoder_inner_cell = keras.layers.LSTMCell(units)
self.decoder_cell = tfa.seq2seq.AttentionWrapper(
cell=decoder_inner_cell,
attention_mechanism=self.attention)
output_layer = keras.layers.Dense(len(OUTPUT_CHARS) + 1)
self.decoder = tfa.seq2seq.BasicDecoder(
cell=self.decoder_cell,
sampler=tfa.seq2seq.sampler.TrainingSampler(),
output_layer=output_layer)
self.inference_decoder = tfa.seq2seq.BasicDecoder(
cell=self.decoder_cell,
sampler=tfa.seq2seq.sampler.GreedyEmbeddingSampler(
embedding_fn=self.decoder_embedding),
output_layer=output_layer,
maximum_iterations=max_output_length)
def call(self, inputs, training=None):
encoder_input, decoder_input = inputs
encoder_embeddings = self.encoder_embedding(encoder_input)
encoder_outputs, encoder_state_h, encoder_state_c = self.encoder(
encoder_embeddings,
training=training)
encoder_state = [encoder_state_h, encoder_state_c]
self.attention(encoder_outputs,
setup_memory=True)
decoder_embeddings = self.decoder_embedding(decoder_input)
decoder_initial_state = self.decoder_cell.get_initial_state(
decoder_embeddings)
decoder_initial_state = decoder_initial_state.clone(
cell_state=encoder_state)
if training:
decoder_outputs, _, _ = self.decoder(
decoder_embeddings,
initial_state=decoder_initial_state,
training=training)
else:
start_tokens = tf.zeros_like(encoder_input[:, 0]) + sos_id
decoder_outputs, _, _ = self.inference_decoder(
decoder_embeddings,
initial_state=decoder_initial_state,
start_tokens=start_tokens,
end_token=0)
return tf.nn.softmax(decoder_outputs.rnn_output)
np.random.seed(42)
tf.random.set_seed(42)
model = DateTranslation()
optimizer = keras.optimizers.Nadam()
model.compile(loss="sparse_categorical_crossentropy", optimizer=optimizer,
metrics=["accuracy"])
history = model.fit([X_train, X_train_decoder], Y_train, epochs=25,
validation_data=([X_valid, X_valid_decoder], Y_valid))
Epoch 1/25
313/313 [==============================] - 7s 21ms/step - loss: 2.1549 - accuracy: 0.2295 - val_loss: 2.1450 - val_accuracy: 0.2239
Epoch 2/25
313/313 [==============================] - 6s 19ms/step - loss: 1.8147 - accuracy: 0.3492 - val_loss: 1.4931 - val_accuracy: 0.4476
Epoch 3/25
313/313 [==============================] - 6s 18ms/step - loss: 1.3585 - accuracy: 0.4909 - val_loss: 1.3168 - val_accuracy: 0.5100
Epoch 4/25
313/313 [==============================] - 6s 18ms/step - loss: 1.2787 - accuracy: 0.5293 - val_loss: 1.1767 - val_accuracy: 0.5624
Epoch 5/25
313/313 [==============================] - 6s 18ms/step - loss: 1.1236 - accuracy: 0.5776 - val_loss: 1.0769 - val_accuracy: 0.5907
Epoch 6/25
313/313 [==============================] - 6s 18ms/step - loss: 1.0369 - accuracy: 0.6073 - val_loss: 1.0159 - val_accuracy: 0.6199
Epoch 7/25
313/313 [==============================] - 6s 18ms/step - loss: 0.9752 - accuracy: 0.6295 - val_loss: 0.9723 - val_accuracy: 0.6346
Epoch 8/25
313/313 [==============================] - 6s 18ms/step - loss: 0.9794 - accuracy: 0.6315 - val_loss: 0.9444 - val_accuracy: 0.6371
Epoch 9/25
313/313 [==============================] - 6s 18ms/step - loss: 0.9338 - accuracy: 0.6415 - val_loss: 0.9296 - val_accuracy: 0.6381
Epoch 10/25
313/313 [==============================] - 6s 19ms/step - loss: 0.9439 - accuracy: 0.6418 - val_loss: 0.9028 - val_accuracy: 0.6574
Epoch 11/25
313/313 [==============================] - 6s 19ms/step - loss: 0.8807 - accuracy: 0.6637 - val_loss: 0.9835 - val_accuracy: 0.6369
Epoch 12/25
313/313 [==============================] - 6s 19ms/step - loss: 0.7307 - accuracy: 0.6953 - val_loss: 0.8942 - val_accuracy: 0.6873
Epoch 13/25
313/313 [==============================] - 6s 19ms/step - loss: 0.5833 - accuracy: 0.7327 - val_loss: 0.6944 - val_accuracy: 0.7391
Epoch 14/25
313/313 [==============================] - 6s 19ms/step - loss: 0.4664 - accuracy: 0.7940 - val_loss: 0.6228 - val_accuracy: 0.7885
Epoch 15/25
313/313 [==============================] - 6s 19ms/step - loss: 0.3205 - accuracy: 0.8740 - val_loss: 0.4825 - val_accuracy: 0.8780
Epoch 16/25
313/313 [==============================] - 6s 19ms/step - loss: 0.2329 - accuracy: 0.9216 - val_loss: 0.3851 - val_accuracy: 0.9118
Epoch 17/25
313/313 [==============================] - 7s 21ms/step - loss: 0.2480 - accuracy: 0.9372 - val_loss: 0.2785 - val_accuracy: 0.9111
Epoch 18/25
313/313 [==============================] - 7s 22ms/step - loss: 0.1182 - accuracy: 0.9801 - val_loss: 0.1372 - val_accuracy: 0.9786
Epoch 19/25
313/313 [==============================] - 7s 22ms/step - loss: 0.0643 - accuracy: 0.9937 - val_loss: 0.0681 - val_accuracy: 0.9909
Epoch 20/25
313/313 [==============================] - 6s 18ms/step - loss: 0.0446 - accuracy: 0.9952 - val_loss: 0.0487 - val_accuracy: 0.9934
Epoch 21/25
313/313 [==============================] - 6s 18ms/step - loss: 0.0247 - accuracy: 0.9987 - val_loss: 0.0228 - val_accuracy: 0.9987
Epoch 22/25
313/313 [==============================] - 6s 18ms/step - loss: 0.0456 - accuracy: 0.9918 - val_loss: 0.0207 - val_accuracy: 0.9985
Epoch 23/25
313/313 [==============================] - 6s 18ms/step - loss: 0.0131 - accuracy: 0.9997 - val_loss: 0.0127 - val_accuracy: 0.9993
Epoch 24/25
313/313 [==============================] - 6s 19ms/step - loss: 0.0360 - accuracy: 0.9933 - val_loss: 0.0146 - val_accuracy: 0.9990
Epoch 25/25
313/313 [==============================] - 6s 19ms/step - loss: 0.0092 - accuracy: 0.9998 - val_loss: 0.0089 - val_accuracy: 0.9992
Not quite 100% validation accuracy, but close. It took a bit longer to converge this time, but there were also more parameters and more computations per iteration. And we did not use a scheduled sampler.
To use the model, we can write yet another little function:
def fast_predict_date_strs_v2(date_strs):
X = prepare_date_strs_padded(date_strs)
X_decoder = tf.zeros(shape=(len(X), max_output_length), dtype=tf.int32)
Y_probas = model.predict([X, X_decoder])
Y_pred = tf.argmax(Y_probas, axis=-1)
return ids_to_date_strs(Y_pred)
fast_predict_date_strs_v2(["July 14, 1789", "May 01, 2020"])
['1789-07-14', '2020-05-01']
There are still a few interesting features from TF-Addons that you may want to look at:
Using a
BeamSearchDecoder
rather than aBasicDecoder
for inference. Instead of outputing the character with the highest probability, this decoder keeps track of the several candidates, and keeps only the most likely sequences of candidates (see chapter 16 in the book for more details).Setting masks or specifying
sequence_length
if the input or target sequences may have very different lengths.Using a
ScheduledOutputTrainingSampler
, which gives you more flexibility than theScheduledEmbeddingTrainingSampler
to decide how to feed the output at time t to the cell at time t+1. By default it feeds the outputs directly to cell, without computing the argmax ID and passing it through an embedding layer. Alternatively, you specify anext_inputs_fn
function that will be used to convert the cell outputs to inputs at the next step.
10.#
Exercise: Go through TensorFlow’s Neural Machine Translation with Attention tutorial.
Simply open the Colab and follow its instructions. Alternatively, if you want a simpler example of using TF-Addons’s seq2seq implementation for Neural Machine Translation (NMT), look at the solution to the previous question. The last model implementation will give you a simpler example of using TF-Addons to build an NMT model using attention mechanisms.
11.#
Exercise: Use one of the recent language models (e.g., GPT) to generate more convincing Shakespearean text.
The simplest way to use recent language models is to use the excellent transformers library, open sourced by Hugging Face. It provides many modern neural net architectures (including BERT, GPT-2, RoBERTa, XLM, DistilBert, XLNet and more) for Natural Language Processing (NLP), including many pretrained models. It relies on either TensorFlow or PyTorch. Best of all: it’s amazingly simple to use.
First, let’s load a pretrained model. In this example, we will use OpenAI’s GPT model, with an additional Language Model on top (just a linear layer with weights tied to the input embeddings). Let’s import it and load the pretrained weights (this will download about 445MB of data to ~/.cache/torch/transformers
):
from transformers import TFOpenAIGPTLMHeadModel
model = TFOpenAIGPTLMHeadModel.from_pretrained("openai-gpt")
Next we will need a specialized tokenizer for this model. This one will try to use the spaCy and ftfy libraries if they are installed, or else it will fall back to BERT’s BasicTokenizer
followed by Byte-Pair Encoding (which should be fine for most use cases).
from transformers import OpenAIGPTTokenizer
tokenizer = OpenAIGPTTokenizer.from_pretrained("openai-gpt")
Now let’s use the tokenizer to tokenize and encode the prompt text:
prompt_text = "This royal throne of kings, this sceptred isle"
encoded_prompt = tokenizer.encode(prompt_text,
add_special_tokens=False,
return_tensors="tf")
encoded_prompt
<tf.Tensor: shape=(1, 10), dtype=int32, numpy=
array([[ 616, 5751, 6404, 498, 9606, 240, 616, 26271, 7428,
16187]], dtype=int32)>
Easy! Next, let’s use the model to generate text after the prompt. We will generate 5 different sentences, each starting with the prompt text, followed by 40 additional tokens. For an explanation of what all the hyperparameters do, make sure to check out this great blog post by Patrick von Platen (from Hugging Face). You can play around with the hyperparameters to try to obtain better results.
num_sequences = 5
length = 40
generated_sequences = model.generate(
input_ids=encoded_prompt,
do_sample=True,
max_length=length + len(encoded_prompt[0]),
temperature=1.0,
top_k=0,
top_p=0.9,
repetition_penalty=1.0,
num_return_sequences=num_sequences,
)
generated_sequences
<tf.Tensor: shape=(5, 50), dtype=int32, numpy=
array([[ 616, 5751, 6404, 498, 9606, 240, 616, 26271, 7428,
16187, 239, 784, 645, 1184, 558, 1886, 688, 6437,
240, 784, 645, 507, 641, 5486, 240, 600, 636,
868, 604, 694, 2816, 485, 1894, 822, 481, 1491,
600, 880, 6061, 239, 256, 40477, 256, 600, 635,
538, 604, 1816, 525, 239],
[ 616, 5751, 6404, 498, 9606, 240, 616, 26271, 7428,
16187, 488, 1288, 989, 640, 16605, 239, 256, 40477,
674, 481, 12744, 3912, 488, 3912, 5936, 2441, 811,
488, 1040, 485, 754, 3952, 239, 40477, 481, 1375,
1981, 833, 1210, 481, 17384, 488, 481, 3089, 488,
481, 4815, 509, 498, 1424],
[ 616, 5751, 6404, 498, 9606, 240, 616, 26271, 7428,
16187, 980, 987, 1074, 13138, 240, 531, 501, 517,
836, 525, 12659, 485, 2642, 512, 239, 500, 616,
7339, 704, 989, 1259, 38752, 481, 9606, 498, 481,
6903, 239, 500, 616, 7339, 704, 3064, 994, 580,
3953, 617, 616, 4741, 488],
[ 616, 5751, 6404, 498, 9606, 240, 616, 26271, 7428,
16187, 10595, 485, 510, 239, 244, 40477, 244, 481,
1424, 6404, 498, 1922, 23, 37492, 257, 244, 40477,
244, 3491, 240, 244, 603, 481, 618, 556, 246,
3386, 498, 524, 756, 239, 244, 616, 1276, 509,
1098, 10945, 498, 246, 6785],
[ 616, 5751, 6404, 498, 9606, 240, 616, 26271, 7428,
16187, 544, 2203, 239, 616, 544, 246, 6460, 260,
850, 629, 4844, 3064, 3766, 240, 246, 1082, 806,
9606, 640, 32581, 240, 595, 7914, 1243, 488, 18535,
239, 249, 587, 538, 788, 775, 2319, 498, 1013,
525, 544, 595, 754, 1074]], dtype=int32)>
Now let’s decode the generated sequences and print them:
for sequence in generated_sequences:
text = tokenizer.decode(sequence, clean_up_tokenization_spaces=True)
print(text)
print("-" * 80)
this royal throne of kings, this sceptred isle. even if someone had given them permission, even if it were required, they would never have been allowed to live through the hell they've survived.'
'they couldn't have known that.
--------------------------------------------------------------------------------
this royal throne of kings, this sceptred isle and these people are royalty.'
then the mute prince and prince edward broke off and went to their rooms.
the talk passed again between the princes and the guards and the princess was of great
--------------------------------------------------------------------------------
this royal throne of kings, this sceptred isle has its own highness, an alatte that waits to save you. in this kingdom your people must emulate the kings of the realm. in this kingdom your kin should be saved from this pit and
--------------------------------------------------------------------------------
this royal throne of kings, this sceptred isle belongs to me. "
" the great throne of penvynne? "
" indeed, " said the king with a nod of his head. " this world was once composed of a magical
--------------------------------------------------------------------------------
this royal throne of kings, this sceptred isle is empty. this is a modern - day fedaykin court, a place where kings are governed, not emperors and judges. i don't see any sign of life that is not their own
--------------------------------------------------------------------------------
You can try more recent (and larger) models, such as GPT-2, CTRL, Transformer-XL or XLNet, which are all available as pretrained models in the transformers library, including variants with Language Models on top. The preprocessing steps vary slightly between models, so make sure to check out this generation example from the transformers documentation (this example uses PyTorch, but it will work with very little tweaks, such as adding TF
at the beginning of the model class name, removing the .to()
method calls, and using return_tensors="tf"
instead of "pt"
.
Hope you enjoyed this chapter! :)