Lab 4.7: Classification Methods#
Original source: emredjan/ISL-python
Added in ISLRv2:
4.7.5 Naive Bayes
4.7.7 Poisson Regression
4.7.1 The Stock Market Data#
import warnings
import numpy as np
import pandas as pd
import matplotlib.pyplot as plt
import seaborn as sns
warnings.filterwarnings("ignore")
%matplotlib inline
pd.set_option('display.max_rows', 12)
pd.set_option('display.float_format', '{:20,.2f}'.format) # get rid of scientific notation
plt.style.use('seaborn') # pretty matplotlib plots
def make_prediction_summary(model, X):
"Generate prediction summary of stock market models using predict_proba method."
return pd.DataFrame(
{
"prob_0": model.predict_proba(X)[:, 0],
"prob_1": model.predict_proba(X)[:, 1],
"pred_class": model.predict(X),
},
columns=["prob_0", "prob_1", "pred_class"],
)
# load data
smarket = pd.read_csv('../datasets/Smarket.csv', index_col=0)
smarket.Direction = smarket.Direction.astype('category')
smarket
Year | Lag1 | Lag2 | Lag3 | Lag4 | Lag5 | Volume | Today | Direction | |
---|---|---|---|---|---|---|---|---|---|
1 | 2001 | 0.38 | -0.19 | -2.62 | -1.05 | 5.01 | 1.19 | 0.96 | Up |
2 | 2001 | 0.96 | 0.38 | -0.19 | -2.62 | -1.05 | 1.30 | 1.03 | Up |
3 | 2001 | 1.03 | 0.96 | 0.38 | -0.19 | -2.62 | 1.41 | -0.62 | Down |
4 | 2001 | -0.62 | 1.03 | 0.96 | 0.38 | -0.19 | 1.28 | 0.61 | Up |
5 | 2001 | 0.61 | -0.62 | 1.03 | 0.96 | 0.38 | 1.21 | 0.21 | Up |
... | ... | ... | ... | ... | ... | ... | ... | ... | ... |
1246 | 2005 | 0.42 | 0.25 | -0.02 | -0.58 | -0.28 | 1.89 | 0.04 | Up |
1247 | 2005 | 0.04 | 0.42 | 0.25 | -0.02 | -0.58 | 1.29 | -0.95 | Down |
1248 | 2005 | -0.95 | 0.04 | 0.42 | 0.25 | -0.02 | 1.54 | 0.13 | Up |
1249 | 2005 | 0.13 | -0.95 | 0.04 | 0.42 | 0.25 | 1.42 | -0.30 | Down |
1250 | 2005 | -0.30 | 0.13 | -0.95 | 0.04 | 0.42 | 1.38 | -0.49 | Down |
1250 rows × 9 columns
smarket.columns
Index(['Year', 'Lag1', 'Lag2', 'Lag3', 'Lag4', 'Lag5', 'Volume', 'Today',
'Direction'],
dtype='object')
smarket.shape
(1250, 9)
smarket.describe(include='all')
Year | Lag1 | Lag2 | Lag3 | Lag4 | Lag5 | Volume | Today | Direction | |
---|---|---|---|---|---|---|---|---|---|
count | 1,250.00 | 1,250.00 | 1,250.00 | 1,250.00 | 1,250.00 | 1,250.00 | 1,250.00 | 1,250.00 | 1250 |
unique | NaN | NaN | NaN | NaN | NaN | NaN | NaN | NaN | 2 |
top | NaN | NaN | NaN | NaN | NaN | NaN | NaN | NaN | Up |
freq | NaN | NaN | NaN | NaN | NaN | NaN | NaN | NaN | 648 |
mean | 2,003.02 | 0.00 | 0.00 | 0.00 | 0.00 | 0.01 | 1.48 | 0.00 | NaN |
std | 1.41 | 1.14 | 1.14 | 1.14 | 1.14 | 1.15 | 0.36 | 1.14 | NaN |
min | 2,001.00 | -4.92 | -4.92 | -4.92 | -4.92 | -4.92 | 0.36 | -4.92 | NaN |
25% | 2,002.00 | -0.64 | -0.64 | -0.64 | -0.64 | -0.64 | 1.26 | -0.64 | NaN |
50% | 2,003.00 | 0.04 | 0.04 | 0.04 | 0.04 | 0.04 | 1.42 | 0.04 | NaN |
75% | 2,004.00 | 0.60 | 0.60 | 0.60 | 0.60 | 0.60 | 1.64 | 0.60 | NaN |
max | 2,005.00 | 5.73 | 5.73 | 5.73 | 5.73 | 5.73 | 3.15 | 5.73 | NaN |
smarket.info(memory_usage='deep')
<class 'pandas.core.frame.DataFrame'>
Index: 1250 entries, 1 to 1250
Data columns (total 9 columns):
# Column Non-Null Count Dtype
--- ------ -------------- -----
0 Year 1250 non-null int64
1 Lag1 1250 non-null float64
2 Lag2 1250 non-null float64
3 Lag3 1250 non-null float64
4 Lag4 1250 non-null float64
5 Lag5 1250 non-null float64
6 Volume 1250 non-null float64
7 Today 1250 non-null float64
8 Direction 1250 non-null category
dtypes: category(1), float64(7), int64(1)
memory usage: 89.3 KB
sns.pairplot(smarket, hue='Direction');
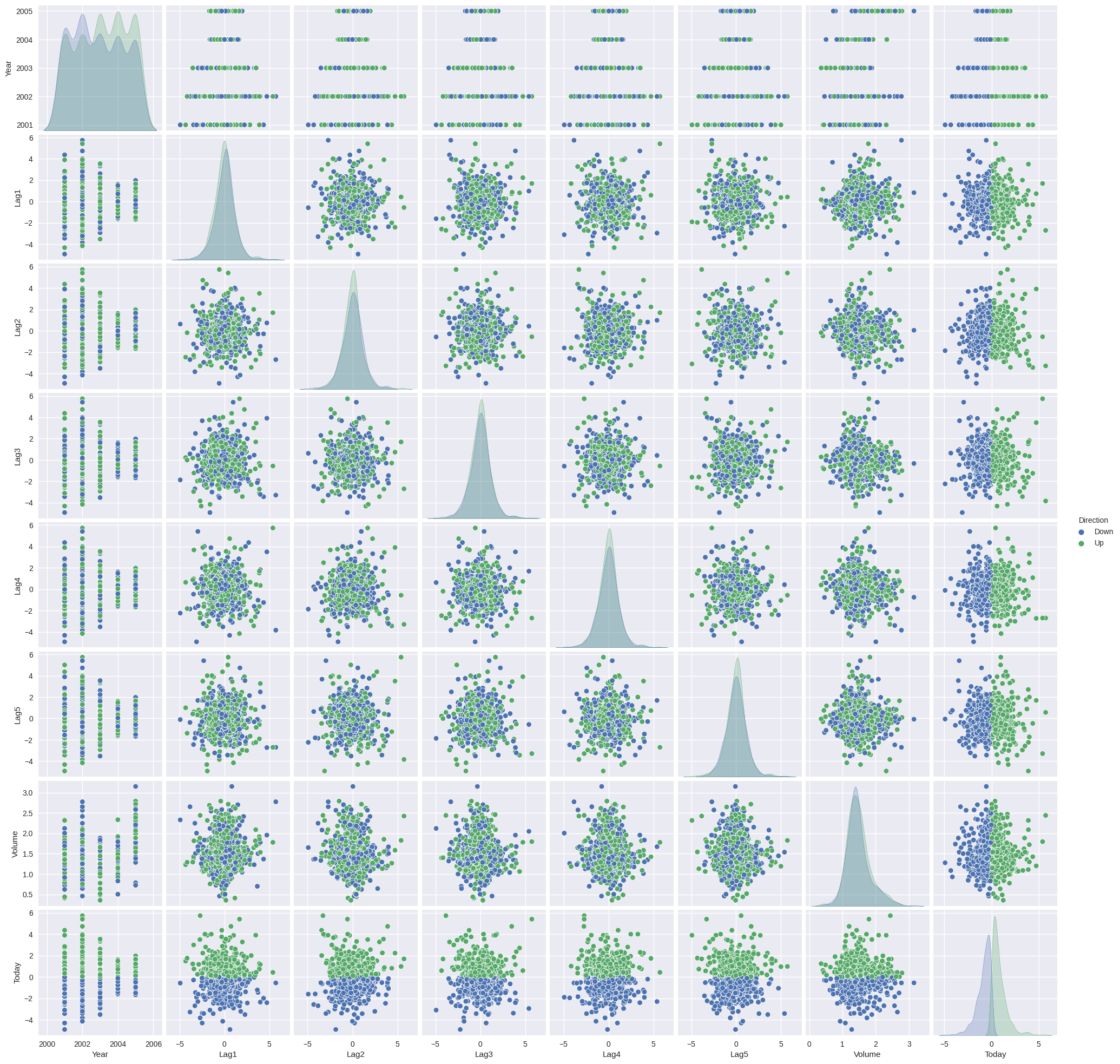
smarket.corr()
---------------------------------------------------------------------------
ValueError Traceback (most recent call last)
Cell In[9], line 1
----> 1 smarket.corr()
File /opt/hostedtoolcache/Python/3.8.18/x64/lib/python3.8/site-packages/pandas/core/frame.py:10054, in DataFrame.corr(self, method, min_periods, numeric_only)
10052 cols = data.columns
10053 idx = cols.copy()
> 10054 mat = data.to_numpy(dtype=float, na_value=np.nan, copy=False)
10056 if method == "pearson":
10057 correl = libalgos.nancorr(mat, minp=min_periods)
File /opt/hostedtoolcache/Python/3.8.18/x64/lib/python3.8/site-packages/pandas/core/frame.py:1838, in DataFrame.to_numpy(self, dtype, copy, na_value)
1836 if dtype is not None:
1837 dtype = np.dtype(dtype)
-> 1838 result = self._mgr.as_array(dtype=dtype, copy=copy, na_value=na_value)
1839 if result.dtype is not dtype:
1840 result = np.array(result, dtype=dtype, copy=False)
File /opt/hostedtoolcache/Python/3.8.18/x64/lib/python3.8/site-packages/pandas/core/internals/managers.py:1732, in BlockManager.as_array(self, dtype, copy, na_value)
1730 arr.flags.writeable = False
1731 else:
-> 1732 arr = self._interleave(dtype=dtype, na_value=na_value)
1733 # The underlying data was copied within _interleave, so no need
1734 # to further copy if copy=True or setting na_value
1736 if na_value is not lib.no_default:
File /opt/hostedtoolcache/Python/3.8.18/x64/lib/python3.8/site-packages/pandas/core/internals/managers.py:1788, in BlockManager._interleave(self, dtype, na_value)
1782 rl = blk.mgr_locs
1783 if blk.is_extension:
1784 # Avoid implicit conversion of extension blocks to object
1785
1786 # error: Item "ndarray" of "Union[ndarray, ExtensionArray]" has no
1787 # attribute "to_numpy"
-> 1788 arr = blk.values.to_numpy( # type: ignore[union-attr]
1789 dtype=dtype,
1790 na_value=na_value,
1791 )
1792 else:
1793 arr = blk.get_values(dtype)
File /opt/hostedtoolcache/Python/3.8.18/x64/lib/python3.8/site-packages/pandas/core/arrays/base.py:485, in ExtensionArray.to_numpy(self, dtype, copy, na_value)
456 def to_numpy(
457 self,
458 dtype: npt.DTypeLike | None = None,
459 copy: bool = False,
460 na_value: object = lib.no_default,
461 ) -> np.ndarray:
462 """
463 Convert to a NumPy ndarray.
464
(...)
483 numpy.ndarray
484 """
--> 485 result = np.asarray(self, dtype=dtype)
486 if copy or na_value is not lib.no_default:
487 result = result.copy()
File /opt/hostedtoolcache/Python/3.8.18/x64/lib/python3.8/site-packages/pandas/core/arrays/_mixins.py:86, in ravel_compat.<locals>.method(self, *args, **kwargs)
83 @wraps(meth)
84 def method(self, *args, **kwargs):
85 if self.ndim == 1:
---> 86 return meth(self, *args, **kwargs)
88 flags = self._ndarray.flags
89 flat = self.ravel("K")
File /opt/hostedtoolcache/Python/3.8.18/x64/lib/python3.8/site-packages/pandas/core/arrays/categorical.py:1344, in Categorical.__array__(self, dtype)
1342 ret = take_nd(self.categories._values, self._codes)
1343 if dtype and not is_dtype_equal(dtype, self.categories.dtype):
-> 1344 return np.asarray(ret, dtype)
1345 # When we're a Categorical[ExtensionArray], like Interval,
1346 # we need to ensure __array__ gets all the way to an
1347 # ndarray.
1348 return np.asarray(ret)
ValueError: could not convert string to float: 'Up'
plt.plot(smarket.Volume);
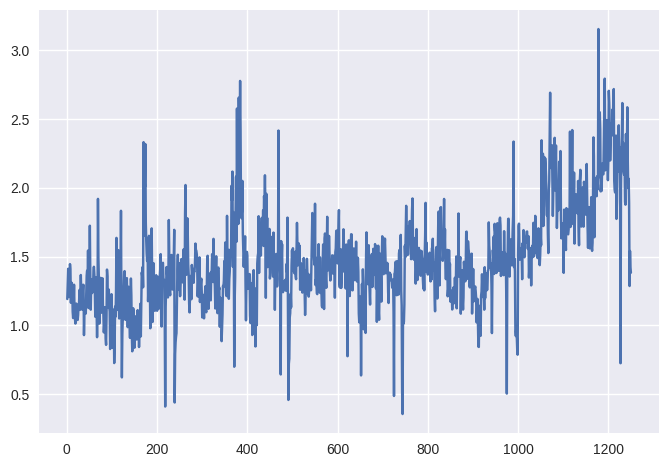
4.7.2 Logistic Regression#
Using statsmodels#
import statsmodels.api as sm
X_sm = smarket.loc[:,'Lag1':'Volume']
y_sm = pd.get_dummies(smarket.Direction).iloc[:, 1] # dummy encoding
glm_fit_sm = sm.Logit(y_sm, sm.add_constant(X_sm)).fit()
glm_fit_sm.summary()
Optimization terminated successfully.
Current function value: 0.691034
Iterations 4
Dep. Variable: | Up | No. Observations: | 1250 |
---|---|---|---|
Model: | Logit | Df Residuals: | 1243 |
Method: | MLE | Df Model: | 6 |
Date: | Sat, 11 Nov 2023 | Pseudo R-squ.: | 0.002074 |
Time: | 11:47:49 | Log-Likelihood: | -863.79 |
converged: | True | LL-Null: | -865.59 |
Covariance Type: | nonrobust | LLR p-value: | 0.7319 |
coef | std err | z | P>|z| | [0.025 | 0.975] | |
---|---|---|---|---|---|---|
const | -0.1260 | 0.241 | -0.523 | 0.601 | -0.598 | 0.346 |
Lag1 | -0.0731 | 0.050 | -1.457 | 0.145 | -0.171 | 0.025 |
Lag2 | -0.0423 | 0.050 | -0.845 | 0.398 | -0.140 | 0.056 |
Lag3 | 0.0111 | 0.050 | 0.222 | 0.824 | -0.087 | 0.109 |
Lag4 | 0.0094 | 0.050 | 0.187 | 0.851 | -0.089 | 0.107 |
Lag5 | 0.0103 | 0.050 | 0.208 | 0.835 | -0.087 | 0.107 |
Volume | 0.1354 | 0.158 | 0.855 | 0.392 | -0.175 | 0.446 |
glm_fit_sm.predict()
array([0.50708413, 0.48146788, 0.48113883, ..., 0.5392683 , 0.52611829,
0.51791656])
glm_fit_sm.pred_table()
array([[145., 457.],
[141., 507.]])
Using scikit-learn#
from sklearn.linear_model import LogisticRegression
X_sk = smarket.loc[:,'Lag1':'Volume']
y_sk = smarket.Direction
glm_model = LogisticRegression(fit_intercept=True, C=1e9) # Large C for no regularization
glm_fit = glm_model.fit(X_sk, y_sk)
glm_fit.intercept_, glm_fit.coef_
(array([-0.12600781]),
array([[-0.07307339, -0.04230074, 0.01108552, 0.00935968, 0.01031339,
0.13544567]]))
make_prediction_summary(glm_fit, X_sk)
prob_0 | prob_1 | pred_class | |
---|---|---|---|
0 | 0.49 | 0.51 | Up |
1 | 0.52 | 0.48 | Down |
2 | 0.52 | 0.48 | Down |
3 | 0.48 | 0.52 | Up |
4 | 0.49 | 0.51 | Up |
... | ... | ... | ... |
1245 | 0.48 | 0.52 | Up |
1246 | 0.49 | 0.51 | Up |
1247 | 0.46 | 0.54 | Up |
1248 | 0.47 | 0.53 | Up |
1249 | 0.48 | 0.52 | Up |
1250 rows × 3 columns
from sklearn.metrics import confusion_matrix, accuracy_score
# labeled confusion matrix for predicted values
conf_m = pd.DataFrame(confusion_matrix(y_sk, glm_fit.predict(X_sk)))
conf_m.columns.name = 'Predicted'
conf_m.index.name = 'True'
conf_m
Predicted | 0 | 1 |
---|---|---|
True | ||
0 | 145 | 457 |
1 | 141 | 507 |
print('Accuracy: ' + str(accuracy_score(y_sk, glm_fit.predict(X_sk))))
print('Training Error: ' + str(1 - accuracy_score(y_sk, glm_fit.predict(X_sk))))
Accuracy: 0.5216
Training Error: 0.47840000000000005
# separate training and test sets
train = smarket.loc[smarket.Year < 2005]
smarket_2005 = smarket.loc[smarket.Year >= 2005]
X_sk_train = train.loc[:,'Lag1':'Volume']
y_sk_train = train.Direction
X_sk_test = smarket_2005.loc[:,'Lag1':'Volume']
y_sk_test = smarket_2005.Direction
glm_model_t = LogisticRegression(fit_intercept=True, C=1e9) # Large C for no regularization
glm_fit_t = glm_model.fit(X_sk_train, y_sk_train)
make_prediction_summary(glm_fit_t, X_sk_test)
prob_0 | prob_1 | pred_class | |
---|---|---|---|
0 | 0.47 | 0.53 | Up |
1 | 0.48 | 0.52 | Up |
2 | 0.48 | 0.52 | Up |
3 | 0.49 | 0.51 | Up |
4 | 0.50 | 0.50 | Down |
... | ... | ... | ... |
247 | 0.52 | 0.48 | Down |
248 | 0.49 | 0.51 | Up |
249 | 0.48 | 0.52 | Up |
250 | 0.48 | 0.52 | Up |
251 | 0.49 | 0.51 | Up |
252 rows × 3 columns
conf_m = pd.DataFrame(confusion_matrix(y_sk_test, glm_fit.predict(X_sk_test)))
conf_m.columns.name = 'Predicted'
conf_m.index.name = 'True'
conf_m
Predicted | 0 | 1 |
---|---|---|
True | ||
0 | 77 | 34 |
1 | 97 | 44 |
print('Accuracy: ' + str(accuracy_score(y_sk_test, glm_fit.predict(X_sk_test))))
print('Test Error: ' + str(1 - accuracy_score(y_sk_test, glm_fit.predict(X_sk_test))))
Accuracy: 0.4801587301587302
Test Error: 0.5198412698412698
X_sk_train_s = train.loc[:,'Lag1':'Lag2']
y_sk_train_s = train.Direction
X_sk_test_s = smarket_2005.loc[:,'Lag1':'Lag2']
y_sk_test_s = smarket_2005.Direction
glm_model_t_s = LogisticRegression(fit_intercept=True, C=1e9) # Large C for no regularization
glm_fit_t_s = glm_model.fit(X_sk_train_s, y_sk_train_s)
make_prediction_summary(glm_fit_t_s, X_sk_test_s)
prob_0 | prob_1 | pred_class | |
---|---|---|---|
0 | 0.49 | 0.51 | Up |
1 | 0.48 | 0.52 | Up |
2 | 0.47 | 0.53 | Up |
3 | 0.47 | 0.53 | Up |
4 | 0.49 | 0.51 | Up |
... | ... | ... | ... |
247 | 0.50 | 0.50 | Down |
248 | 0.50 | 0.50 | Up |
249 | 0.48 | 0.52 | Up |
250 | 0.48 | 0.52 | Up |
251 | 0.49 | 0.51 | Up |
252 rows × 3 columns
conf_m = pd.DataFrame(confusion_matrix(y_sk_test_s, glm_fit.predict(X_sk_test_s)))
conf_m.columns.name = 'Predicted'
conf_m.index.name = 'True'
conf_m
Predicted | 0 | 1 |
---|---|---|
True | ||
0 | 35 | 76 |
1 | 35 | 106 |
print('Accuracy: ' + str(accuracy_score(y_sk_test_s, glm_fit.predict(X_sk_test_s))))
print('Test Error: ' + str(1 - accuracy_score(y_sk_test_s, glm_fit.predict(X_sk_test_s))))
Accuracy: 0.5595238095238095
Test Error: 0.44047619047619047
# predictions for arbitrary data
predict_data = pd.DataFrame({'Lag1': [1.2, 1.5], 'Lag2': [1.1, -0.8]})
glm_fit.predict_proba(predict_data)[:, 1]
array([0.47914604, 0.49611109])
4.7.3 Linear Discriminant Analysis#
from sklearn.discriminant_analysis import LinearDiscriminantAnalysis
X_train = train.loc[:,'Lag1':'Lag2']
y_train = train.Direction
X_test = smarket_2005.loc[:,'Lag1':'Lag2']
y_test = smarket_2005.Direction
lda_model = LinearDiscriminantAnalysis()
lda_fit = lda_model.fit(X_train, y_train)
lda_fit.priors_ # group priors
array([0.49198397, 0.50801603])
lda_fit.means_ # group means
array([[ 0.04279022, 0.03389409],
[-0.03954635, -0.03132544]])
lda_fit.scalings_ # coefficients of ld
array([[-0.64201904],
[-0.51352928]])
# for plotting lda, needs imporovement
ld_sc = X_train.iloc[:, 0] * lda_fit.scalings_[0] + X_train.iloc[:, 1] * lda_fit.scalings_[1]
ld = pd.DataFrame({'groups': y_train, 'ld': ld_sc})
g = sns.FacetGrid(ld, col='groups')
g.map(plt.hist, 'ld');
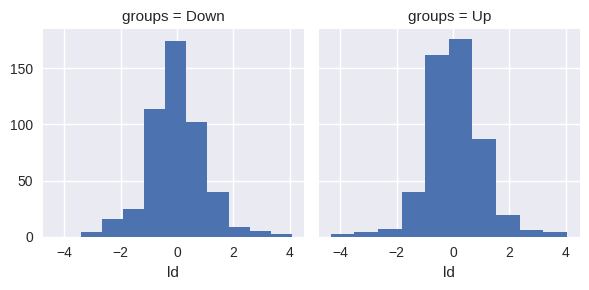
# prediction summary
pd.DataFrame({'prob_0': lda_fit.predict_proba(X_test)[:, 0],
'prob_1': lda_fit.predict_proba(X_test)[:, 1],
'pred_class': lda_fit.predict(X_test)},
columns=['prob_0', 'prob_1', 'pred_class'])
prob_0 | prob_1 | pred_class | |
---|---|---|---|
0 | 0.49 | 0.51 | Up |
1 | 0.48 | 0.52 | Up |
2 | 0.47 | 0.53 | Up |
3 | 0.47 | 0.53 | Up |
4 | 0.49 | 0.51 | Up |
... | ... | ... | ... |
247 | 0.50 | 0.50 | Down |
248 | 0.50 | 0.50 | Up |
249 | 0.48 | 0.52 | Up |
250 | 0.48 | 0.52 | Up |
251 | 0.49 | 0.51 | Up |
252 rows × 3 columns
conf_m = pd.DataFrame(confusion_matrix(y_test, glm_fit.predict(X_test)))
conf_m.columns.name = 'Predicted'
conf_m.index.name = 'True'
conf_m
Predicted | 0 | 1 |
---|---|---|
True | ||
0 | 35 | 76 |
1 | 35 | 106 |
4.7.4 Quadratic Discriminant Analysis#
from sklearn.discriminant_analysis import QuadraticDiscriminantAnalysis
X_train = train.loc[:,'Lag1':'Lag2']
y_train = train.Direction
X_test = smarket_2005.loc[:,'Lag1':'Lag2']
y_test = smarket_2005.Direction
qda_model = QuadraticDiscriminantAnalysis()
qda_fit = qda_model.fit(X_train, y_train)
qda_fit.priors_ # group priors
array([0.49198397, 0.50801603])
qda_fit.means_ # group means
array([[ 0.04279022, 0.03389409],
[-0.03954635, -0.03132544]])
qda_fit.scalings_ # coefficients of ld
[array([1.56294495, 1.47927279]), array([1.53455065, 1.47272326])]
# prediction summary
pd.DataFrame({'prob_0': qda_fit.predict_proba(X_test)[:, 0],
'prob_1': qda_fit.predict_proba(X_test)[:, 1],
'pred_class': qda_fit.predict(X_test)},
columns=['prob_0', 'prob_1', 'pred_class'])
prob_0 | prob_1 | pred_class | |
---|---|---|---|
0 | 0.49 | 0.51 | Up |
1 | 0.48 | 0.52 | Up |
2 | 0.46 | 0.54 | Up |
3 | 0.47 | 0.53 | Up |
4 | 0.49 | 0.51 | Up |
... | ... | ... | ... |
247 | 0.50 | 0.50 | Up |
248 | 0.49 | 0.51 | Up |
249 | 0.48 | 0.52 | Up |
250 | 0.48 | 0.52 | Up |
251 | 0.49 | 0.51 | Up |
252 rows × 3 columns
conf_m = pd.DataFrame(confusion_matrix(y_test, qda_fit.predict(X_test)))
conf_m.columns.name = 'Predicted'
conf_m.index.name = 'True'
conf_m
Predicted | 0 | 1 |
---|---|---|
True | ||
0 | 30 | 81 |
1 | 20 | 121 |
print(f'Accuracy: {accuracy_score(y_test, qda_fit.predict(X_test)):.3f}')
print(f'Test Error: {1 - accuracy_score(y_test, qda_fit.predict(X_test)):.3f}')
Accuracy: 0.599
Test Error: 0.401
4.7.5 Naive Bayes#
from sklearn.naive_bayes import GaussianNB
X_train = train.loc[:,'Lag1':'Lag2']
y_train = train.Direction
X_test = smarket_2005.loc[:,'Lag1':'Lag2']
y_test = smarket_2005.Direction
nb_model = GaussianNB()
nb_fit = nb_model.fit(X_train, y_train)
make_prediction_summary(nb_fit, X_test)
prob_0 | prob_1 | pred_class | |
---|---|---|---|
0 | 0.49 | 0.51 | Up |
1 | 0.48 | 0.52 | Up |
2 | 0.47 | 0.53 | Up |
3 | 0.47 | 0.53 | Up |
4 | 0.49 | 0.51 | Up |
... | ... | ... | ... |
247 | 0.50 | 0.50 | Up |
248 | 0.49 | 0.51 | Up |
249 | 0.48 | 0.52 | Up |
250 | 0.48 | 0.52 | Up |
251 | 0.49 | 0.51 | Up |
252 rows × 3 columns
print(f'Accuracy: {accuracy_score(y_test, nb_fit.predict(X_test)):.3f}')
print(f'Test Error: {1 - accuracy_score(y_test, nb_fit.predict(X_test)):.3f}')
Accuracy: 0.595
Test Error: 0.405
4.7.6 K-Nearest Neighbors#
Stock market data#
from sklearn.neighbors import KNeighborsClassifier
X_train = train.loc[:,'Lag1':'Lag2']
y_train = train.Direction
X_test = smarket_2005.loc[:,'Lag1':'Lag2']
y_test = smarket_2005.Direction
knn_model = KNeighborsClassifier(n_neighbors=1)
knn_fit = knn_model.fit(X_train, y_train)
make_prediction_summary(knn_fit, X_test)
prob_0 | prob_1 | pred_class | |
---|---|---|---|
0 | 0.00 | 1.00 | Up |
1 | 1.00 | 0.00 | Down |
2 | 0.00 | 1.00 | Up |
3 | 0.00 | 1.00 | Up |
4 | 0.00 | 1.00 | Up |
... | ... | ... | ... |
247 | 1.00 | 0.00 | Down |
248 | 1.00 | 0.00 | Down |
249 | 0.00 | 1.00 | Up |
250 | 1.00 | 0.00 | Down |
251 | 0.00 | 1.00 | Up |
252 rows × 3 columns
conf_m = pd.DataFrame(confusion_matrix(y_test, knn_fit.predict(X_test)))
conf_m.columns.name = 'Predicted'
conf_m.index.name = 'True'
conf_m
Predicted | 0 | 1 |
---|---|---|
True | ||
0 | 43 | 68 |
1 | 58 | 83 |
print('Accuracy: ' + str(accuracy_score(y_test, knn_fit.predict(X_test))))
print('Test Error: ' + str(1 - accuracy_score(y_test, knn_fit.predict(X_test))))
Accuracy: 0.5
Test Error: 0.5
knn_model_3 = KNeighborsClassifier(n_neighbors=3)
knn_fit_3 = knn_model_3.fit(X_train, y_train)
conf_m = pd.DataFrame(confusion_matrix(y_test, knn_fit_3.predict(X_test)))
conf_m.columns.name = 'Predicted'
conf_m.index.name = 'True'
conf_m
Predicted | 0 | 1 |
---|---|---|
True | ||
0 | 48 | 63 |
1 | 55 | 86 |
print(f'Accuracy: {accuracy_score(y_test, knn_fit_3.predict(X_test)):.3f}')
print(f'Test Error: {1 - accuracy_score(y_test, knn_fit_3.predict(X_test)):.3f}')
Accuracy: 0.532
Test Error: 0.468
Caravan insurance data#
# load data
caravan = pd.read_csv('../datasets/Caravan.csv', index_col=0)
caravan.Purchase = caravan.Purchase.astype('category')
caravan
MOSTYPE | MAANTHUI | MGEMOMV | MGEMLEEF | MOSHOOFD | MGODRK | MGODPR | MGODOV | MGODGE | MRELGE | ... | APERSONG | AGEZONG | AWAOREG | ABRAND | AZEILPL | APLEZIER | AFIETS | AINBOED | ABYSTAND | Purchase | |
---|---|---|---|---|---|---|---|---|---|---|---|---|---|---|---|---|---|---|---|---|---|
1 | 33 | 1 | 3 | 2 | 8 | 0 | 5 | 1 | 3 | 7 | ... | 0 | 0 | 0 | 1 | 0 | 0 | 0 | 0 | 0 | No |
2 | 37 | 1 | 2 | 2 | 8 | 1 | 4 | 1 | 4 | 6 | ... | 0 | 0 | 0 | 1 | 0 | 0 | 0 | 0 | 0 | No |
3 | 37 | 1 | 2 | 2 | 8 | 0 | 4 | 2 | 4 | 3 | ... | 0 | 0 | 0 | 1 | 0 | 0 | 0 | 0 | 0 | No |
4 | 9 | 1 | 3 | 3 | 3 | 2 | 3 | 2 | 4 | 5 | ... | 0 | 0 | 0 | 1 | 0 | 0 | 0 | 0 | 0 | No |
5 | 40 | 1 | 4 | 2 | 10 | 1 | 4 | 1 | 4 | 7 | ... | 0 | 0 | 0 | 1 | 0 | 0 | 0 | 0 | 0 | No |
... | ... | ... | ... | ... | ... | ... | ... | ... | ... | ... | ... | ... | ... | ... | ... | ... | ... | ... | ... | ... | ... |
5818 | 36 | 1 | 1 | 2 | 8 | 0 | 6 | 1 | 2 | 1 | ... | 0 | 0 | 0 | 1 | 0 | 0 | 0 | 0 | 0 | No |
5819 | 35 | 1 | 4 | 4 | 8 | 1 | 4 | 1 | 4 | 6 | ... | 0 | 0 | 0 | 1 | 0 | 0 | 0 | 0 | 0 | No |
5820 | 33 | 1 | 3 | 4 | 8 | 0 | 6 | 0 | 3 | 5 | ... | 0 | 0 | 0 | 1 | 0 | 0 | 0 | 0 | 0 | Yes |
5821 | 34 | 1 | 3 | 2 | 8 | 0 | 7 | 0 | 2 | 7 | ... | 0 | 0 | 0 | 0 | 0 | 0 | 0 | 0 | 0 | No |
5822 | 33 | 1 | 3 | 3 | 8 | 0 | 6 | 1 | 2 | 7 | ... | 0 | 0 | 0 | 0 | 0 | 0 | 0 | 0 | 0 | No |
5822 rows × 86 columns
caravan.info(memory_usage='deep')
<class 'pandas.core.frame.DataFrame'>
Index: 5822 entries, 1 to 5822
Data columns (total 86 columns):
# Column Non-Null Count Dtype
--- ------ -------------- -----
0 MOSTYPE 5822 non-null int64
1 MAANTHUI 5822 non-null int64
2 MGEMOMV 5822 non-null int64
3 MGEMLEEF 5822 non-null int64
4 MOSHOOFD 5822 non-null int64
5 MGODRK 5822 non-null int64
6 MGODPR 5822 non-null int64
7 MGODOV 5822 non-null int64
8 MGODGE 5822 non-null int64
9 MRELGE 5822 non-null int64
10 MRELSA 5822 non-null int64
11 MRELOV 5822 non-null int64
12 MFALLEEN 5822 non-null int64
13 MFGEKIND 5822 non-null int64
14 MFWEKIND 5822 non-null int64
15 MOPLHOOG 5822 non-null int64
16 MOPLMIDD 5822 non-null int64
17 MOPLLAAG 5822 non-null int64
18 MBERHOOG 5822 non-null int64
19 MBERZELF 5822 non-null int64
20 MBERBOER 5822 non-null int64
21 MBERMIDD 5822 non-null int64
22 MBERARBG 5822 non-null int64
23 MBERARBO 5822 non-null int64
24 MSKA 5822 non-null int64
25 MSKB1 5822 non-null int64
26 MSKB2 5822 non-null int64
27 MSKC 5822 non-null int64
28 MSKD 5822 non-null int64
29 MHHUUR 5822 non-null int64
30 MHKOOP 5822 non-null int64
31 MAUT1 5822 non-null int64
32 MAUT2 5822 non-null int64
33 MAUT0 5822 non-null int64
34 MZFONDS 5822 non-null int64
35 MZPART 5822 non-null int64
36 MINKM30 5822 non-null int64
37 MINK3045 5822 non-null int64
38 MINK4575 5822 non-null int64
39 MINK7512 5822 non-null int64
40 MINK123M 5822 non-null int64
41 MINKGEM 5822 non-null int64
42 MKOOPKLA 5822 non-null int64
43 PWAPART 5822 non-null int64
44 PWABEDR 5822 non-null int64
45 PWALAND 5822 non-null int64
46 PPERSAUT 5822 non-null int64
47 PBESAUT 5822 non-null int64
48 PMOTSCO 5822 non-null int64
49 PVRAAUT 5822 non-null int64
50 PAANHANG 5822 non-null int64
51 PTRACTOR 5822 non-null int64
52 PWERKT 5822 non-null int64
53 PBROM 5822 non-null int64
54 PLEVEN 5822 non-null int64
55 PPERSONG 5822 non-null int64
56 PGEZONG 5822 non-null int64
57 PWAOREG 5822 non-null int64
58 PBRAND 5822 non-null int64
59 PZEILPL 5822 non-null int64
60 PPLEZIER 5822 non-null int64
61 PFIETS 5822 non-null int64
62 PINBOED 5822 non-null int64
63 PBYSTAND 5822 non-null int64
64 AWAPART 5822 non-null int64
65 AWABEDR 5822 non-null int64
66 AWALAND 5822 non-null int64
67 APERSAUT 5822 non-null int64
68 ABESAUT 5822 non-null int64
69 AMOTSCO 5822 non-null int64
70 AVRAAUT 5822 non-null int64
71 AAANHANG 5822 non-null int64
72 ATRACTOR 5822 non-null int64
73 AWERKT 5822 non-null int64
74 ABROM 5822 non-null int64
75 ALEVEN 5822 non-null int64
76 APERSONG 5822 non-null int64
77 AGEZONG 5822 non-null int64
78 AWAOREG 5822 non-null int64
79 ABRAND 5822 non-null int64
80 AZEILPL 5822 non-null int64
81 APLEZIER 5822 non-null int64
82 AFIETS 5822 non-null int64
83 AINBOED 5822 non-null int64
84 ABYSTAND 5822 non-null int64
85 Purchase 5822 non-null category
dtypes: category(1), int64(85)
memory usage: 3.8 MB
caravan.Purchase.value_counts()
Purchase
No 5474
Yes 348
Name: count, dtype: int64
caravan.Purchase.value_counts()['Yes']/len(caravan)
0.05977327378907592
from scipy.stats import zscore
X = caravan.iloc[:, 0:85].apply(zscore) # standardize for KNN
y = caravan.Purchase
X_train = X.loc[1001:]
y_train = y.loc[1001:]
X_test = X.loc[:1000]
y_test = y.loc[:1000]
knn_model = KNeighborsClassifier(n_neighbors=1)
knn_fit = knn_model.fit(X_train, y_train)
conf_m = pd.DataFrame(confusion_matrix(y_test, knn_fit.predict(X_test)))
conf_m.columns.name = 'Predicted'
conf_m.index.name = 'True'
conf_m
Predicted | 0 | 1 |
---|---|---|
True | ||
0 | 873 | 68 |
1 | 50 | 9 |
print(f'Accuracy: {accuracy_score(y_test, knn_fit.predict(X_test)):.3f}')
print(f'Test Error: {1 - accuracy_score(y_test, knn_fit.predict(X_test)):.3f}')
Accuracy: 0.882
Test Error: 0.118
from sklearn.metrics import precision_score
print('Precision for \'Yes\': ' + str(precision_score(y_test, knn_fit.predict(X_test), pos_label='Yes')))
Precision for 'Yes': 0.11688311688311688
knn_model = KNeighborsClassifier(n_neighbors=3)
knn_fit3 = knn_model.fit(X_train, y_train)
print('Precision for \'Yes\': ' + str(precision_score(y_test, knn_fit3.predict(X_test), pos_label='Yes')))
Precision for 'Yes': 0.2
knn_model = KNeighborsClassifier(n_neighbors=5)
knn_fit5 = knn_model.fit(X_train, y_train)
print('Precision for \'Yes\': ' + str(precision_score(y_test, knn_fit5.predict(X_test), pos_label='Yes')))
Precision for 'Yes': 0.2857142857142857
glm_model = LogisticRegression(fit_intercept=True, C=1e9) # Large C for no regularization
glm_fit = glm_model.fit(X_train, y_train)
glm_pred_50 = pd.Series(glm_fit.predict_proba(X_test)[:, 1] > 0.5).map({False: 'No', True: 'Yes'})
conf_m = pd.DataFrame(confusion_matrix(y_test, glm_pred_50))
conf_m.columns.name = 'Predicted'
conf_m.index.name = 'True'
conf_m
Predicted | 0 | 1 |
---|---|---|
True | ||
0 | 935 | 6 |
1 | 59 | 0 |
print('Precision for \'Yes\': ' + str(precision_score(y_test, glm_pred_50, pos_label='Yes')))
Precision for 'Yes': 0.0
glm_pred_25 = pd.Series(glm_fit.predict_proba(X_test)[:, 1] > 0.25).map({False: 'No', True: 'Yes'})
conf_m = pd.DataFrame(confusion_matrix(y_test, glm_pred_25))
conf_m.columns.name = 'Predicted'
conf_m.index.name = 'True'
conf_m
Predicted | 0 | 1 |
---|---|---|
True | ||
0 | 918 | 23 |
1 | 48 | 11 |
print('Precision for \'Yes\': ' + str(precision_score(y_test, glm_pred_25, pos_label='Yes')))
Precision for 'Yes': 0.3235294117647059