Lab 3.6: Linear Regression#
3.6.2 Simple Linear Regression#
import warnings
import numpy as np
import matplotlib.pyplot as plt
import pandas as pd
import seaborn as sns
warnings.filterwarnings("ignore")
%matplotlib inline
pd.set_option('display.max_rows', 10)
pd.set_option('display.float_format', '{:20,.2f}'.format) # get rid of scientific notation
plt.style.use('seaborn') # pretty matplotlib plots
# load data
boston = pd.read_csv('../datasets/Boston.csv', index_col=0)
boston
crim | zn | indus | chas | nox | rm | age | dis | rad | tax | ptratio | black | lstat | medv | |
---|---|---|---|---|---|---|---|---|---|---|---|---|---|---|
1 | 0.01 | 18.00 | 2.31 | 0 | 0.54 | 6.58 | 65.20 | 4.09 | 1 | 296 | 15.30 | 396.90 | 4.98 | 24.00 |
2 | 0.03 | 0.00 | 7.07 | 0 | 0.47 | 6.42 | 78.90 | 4.97 | 2 | 242 | 17.80 | 396.90 | 9.14 | 21.60 |
3 | 0.03 | 0.00 | 7.07 | 0 | 0.47 | 7.18 | 61.10 | 4.97 | 2 | 242 | 17.80 | 392.83 | 4.03 | 34.70 |
4 | 0.03 | 0.00 | 2.18 | 0 | 0.46 | 7.00 | 45.80 | 6.06 | 3 | 222 | 18.70 | 394.63 | 2.94 | 33.40 |
5 | 0.07 | 0.00 | 2.18 | 0 | 0.46 | 7.15 | 54.20 | 6.06 | 3 | 222 | 18.70 | 396.90 | 5.33 | 36.20 |
... | ... | ... | ... | ... | ... | ... | ... | ... | ... | ... | ... | ... | ... | ... |
502 | 0.06 | 0.00 | 11.93 | 0 | 0.57 | 6.59 | 69.10 | 2.48 | 1 | 273 | 21.00 | 391.99 | 9.67 | 22.40 |
503 | 0.05 | 0.00 | 11.93 | 0 | 0.57 | 6.12 | 76.70 | 2.29 | 1 | 273 | 21.00 | 396.90 | 9.08 | 20.60 |
504 | 0.06 | 0.00 | 11.93 | 0 | 0.57 | 6.98 | 91.00 | 2.17 | 1 | 273 | 21.00 | 396.90 | 5.64 | 23.90 |
505 | 0.11 | 0.00 | 11.93 | 0 | 0.57 | 6.79 | 89.30 | 2.39 | 1 | 273 | 21.00 | 393.45 | 6.48 | 22.00 |
506 | 0.05 | 0.00 | 11.93 | 0 | 0.57 | 6.03 | 80.80 | 2.50 | 1 | 273 | 21.00 | 396.90 | 7.88 | 11.90 |
506 rows × 14 columns
Using scikit-learn#
from sklearn import linear_model
# ols model with intercept
ols_sl = linear_model.LinearRegression(fit_intercept=True)
# fitted ols model (.values.reshape(-1, 1) is required for single predictor?)
x_train = boston['lstat'].values.reshape(-1, 1)
y_true = boston['medv']
ols_sl.fit(x_train, y_true)
# summary
ols_sl.intercept_, ols_sl.coef_
(34.5538408793831, array([-0.95004935]))
# metrics
from sklearn.metrics import mean_squared_error, explained_variance_score, r2_score
y_pred = ols_sl.predict(boston['lstat'].values.reshape(-1, 1))
ols_sl_summary = {'R2': r2_score(y_true, y_pred),
'Ex. Var': explained_variance_score(y_true, y_pred),
'MSE': mean_squared_error(y_true, y_pred)}
for k, v in ols_sl_summary.items():
print(k, ':', v)
R2 : 0.5441462975864797
Ex. Var : 0.5441462975864798
MSE : 38.48296722989415
# out-of-sample predictions
ols_sl.predict(np.array([5, 10, 15]).reshape(-1, 1))
array([29.80359411, 25.05334734, 20.30310057])
Using statsmodels#
# using statsmodels
import statsmodels.api as sm
# predictor & dependent var
x_train = boston['lstat']
y_true = boston['medv']
# ols model with intercept added to predictor
ols_sm = sm.OLS(y_true, sm.add_constant(x_train))
# fitted model and summary
ols_sm_results = ols_sm.fit()
ols_sm_results.summary()
# robust SE
#ols_sm_robust = sm.RLM(boston['medv'], X, M=sm.robust.norms.LeastSquares())
#ols_sm_robust.fit(cov='H2').summary()
Dep. Variable: | medv | R-squared: | 0.544 |
---|---|---|---|
Model: | OLS | Adj. R-squared: | 0.543 |
Method: | Least Squares | F-statistic: | 601.6 |
Date: | Sat, 11 Nov 2023 | Prob (F-statistic): | 5.08e-88 |
Time: | 11:47:24 | Log-Likelihood: | -1641.5 |
No. Observations: | 506 | AIC: | 3287. |
Df Residuals: | 504 | BIC: | 3295. |
Df Model: | 1 | ||
Covariance Type: | nonrobust |
coef | std err | t | P>|t| | [0.025 | 0.975] | |
---|---|---|---|---|---|---|
const | 34.5538 | 0.563 | 61.415 | 0.000 | 33.448 | 35.659 |
lstat | -0.9500 | 0.039 | -24.528 | 0.000 | -1.026 | -0.874 |
Omnibus: | 137.043 | Durbin-Watson: | 0.892 |
---|---|---|---|
Prob(Omnibus): | 0.000 | Jarque-Bera (JB): | 291.373 |
Skew: | 1.453 | Prob(JB): | 5.36e-64 |
Kurtosis: | 5.319 | Cond. No. | 29.7 |
Notes:
[1] Standard Errors assume that the covariance matrix of the errors is correctly specified.
# out-of-sample predictions
ols_sm_results.predict(sm.add_constant([5, 10, 15]))
array([29.80359411, 25.05334734, 20.30310057])
# prediction intervals
from statsmodels.sandbox.regression.predstd import wls_prediction_std
from collections import OrderedDict
y_pred = ols_sm_results.predict(sm.add_constant(x_train))
prstd, iv_l, iv_u = wls_prediction_std(ols_sm_results)
pred_dict = OrderedDict({'x_train': x_train,
'y_pred': y_pred,
'y_true': y_true,
'lwr': iv_l,
'upr': iv_u,
'pred_se': prstd})
pd.DataFrame(pred_dict)
x_train | y_pred | y_true | lwr | upr | pred_se | |
---|---|---|---|---|---|---|
1 | 4.98 | 29.82 | 24.00 | 17.58 | 42.06 | 6.23 |
2 | 9.14 | 25.87 | 21.60 | 13.64 | 38.10 | 6.22 |
3 | 4.03 | 30.73 | 34.70 | 18.48 | 42.97 | 6.23 |
4 | 2.94 | 31.76 | 33.40 | 19.51 | 44.01 | 6.23 |
5 | 5.33 | 29.49 | 36.20 | 17.25 | 41.73 | 6.23 |
... | ... | ... | ... | ... | ... | ... |
502 | 9.67 | 25.37 | 22.40 | 13.14 | 37.59 | 6.22 |
503 | 9.08 | 25.93 | 20.60 | 13.70 | 38.15 | 6.22 |
504 | 5.64 | 29.20 | 23.90 | 16.96 | 41.43 | 6.23 |
505 | 6.48 | 28.40 | 22.00 | 16.16 | 40.63 | 6.23 |
506 | 7.88 | 27.07 | 11.90 | 14.84 | 39.30 | 6.22 |
506 rows × 6 columns
sns.regplot('lstat', 'medv', data=boston);
---------------------------------------------------------------------------
TypeError Traceback (most recent call last)
Cell In[9], line 1
----> 1 sns.regplot('lstat', 'medv', data=boston);
TypeError: regplot() got multiple values for argument 'data'
from statsmodels.graphics.regressionplots import *
ols_sm_resid = ols_sm_results.resid # residuals
ols_sm_resid_stud = ols_sm_resid / prstd # studentized residuals
f, axes = plt.subplots(2, 2, sharex=False, sharey=False)
f.set_figheight(10)
f.set_figwidth(15)
sns.regplot('lstat', 'medv', data=boston, ax=axes[0, 0], scatter_kws={'alpha': 0.5}) # regression plot
sns.residplot(y_pred, 'medv', data=boston, ax=axes[0, 1], scatter_kws={'alpha': 0.5}) # residual plot
#plot_leverage_resid2(ols_sm_results, ax=axes[1, 0], color='red') # leverage plot
# custom leverage plot instead of above
from statsmodels.stats.outliers_influence import OLSInfluence
from scipy.stats import zscore
norm_resid = zscore(ols_sm_resid)
leverage = OLSInfluence(ols_sm_results).hat_matrix_diag
axes[1, 0].autoscale(enable=True, axis='y', tight=True)
axes[1, 0].scatter(norm_resid ** 2, leverage, alpha=0.5, color='red')
# studentized residual plot
axes[1, 1].scatter(y_pred, ols_sm_resid_stud, alpha=0.5, color='magenta')
axes[1, 1].axhline(0, ls=":", c=".2")
axes[1, 1].axhline(-3, ls=":", c=".6")
axes[1, 1].axhline(3, ls=":", c=".6")
axes[1, 1].set_ylim(-5, 5)
x = y_pred[np.logical_or(ols_sm_resid_stud > 3, ols_sm_resid_stud < -3)]
y = ols_sm_resid_stud[np.logical_or(ols_sm_resid_stud > 3, ols_sm_resid_stud < -3)]
for i, x, y in zip(x.index, x, y):
axes[1, 1].annotate(i, xy=(x, y));
---------------------------------------------------------------------------
TypeError Traceback (most recent call last)
Cell In[10], line 10
7 f.set_figheight(10)
8 f.set_figwidth(15)
---> 10 sns.regplot('lstat', 'medv', data=boston, ax=axes[0, 0], scatter_kws={'alpha': 0.5}) # regression plot
11 sns.residplot(y_pred, 'medv', data=boston, ax=axes[0, 1], scatter_kws={'alpha': 0.5}) # residual plot
13 #plot_leverage_resid2(ols_sm_results, ax=axes[1, 0], color='red') # leverage plot
14
15 # custom leverage plot instead of above
TypeError: regplot() got multiple values for argument 'data'
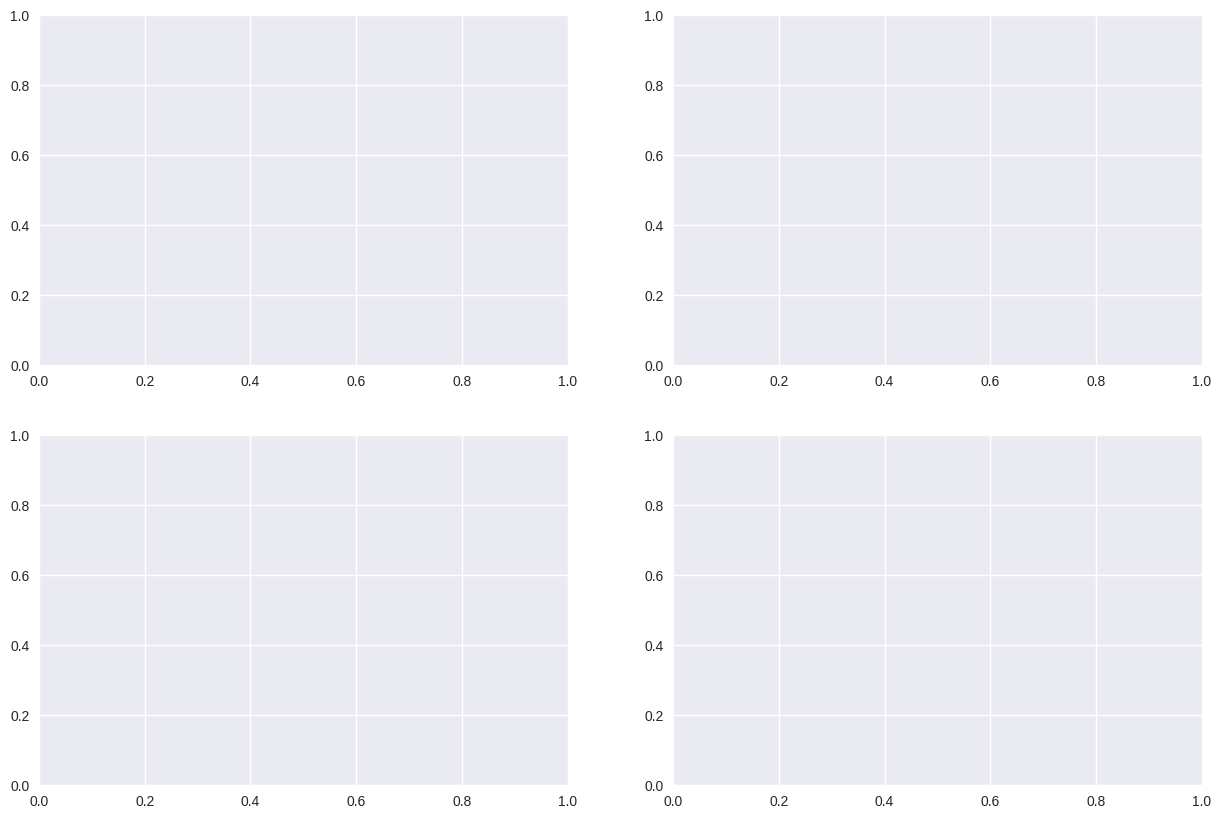
# item with the highest leverage (0-indexed)
leverage.argmax(), leverage.max()
---------------------------------------------------------------------------
NameError Traceback (most recent call last)
Cell In[11], line 3
1 # item with the highest leverage (0-indexed)
----> 3 leverage.argmax(), leverage.max()
NameError: name 'leverage' is not defined
3.6.3 Multiple Linear Regression#
# predictors & dependent var
x_train = boston[['lstat', 'age']]
y_true = boston['medv']
# ols model with intercept added to predictor
ols_sm = sm.OLS(y_true, sm.add_constant(x_train))
# fitted model and summary
ols_sm_results = ols_sm.fit()
ols_sm_results.summary()
Dep. Variable: | medv | R-squared: | 0.551 |
---|---|---|---|
Model: | OLS | Adj. R-squared: | 0.549 |
Method: | Least Squares | F-statistic: | 309.0 |
Date: | Sat, 11 Nov 2023 | Prob (F-statistic): | 2.98e-88 |
Time: | 11:47:25 | Log-Likelihood: | -1637.5 |
No. Observations: | 506 | AIC: | 3281. |
Df Residuals: | 503 | BIC: | 3294. |
Df Model: | 2 | ||
Covariance Type: | nonrobust |
coef | std err | t | P>|t| | [0.025 | 0.975] | |
---|---|---|---|---|---|---|
const | 33.2228 | 0.731 | 45.458 | 0.000 | 31.787 | 34.659 |
lstat | -1.0321 | 0.048 | -21.416 | 0.000 | -1.127 | -0.937 |
age | 0.0345 | 0.012 | 2.826 | 0.005 | 0.011 | 0.059 |
Omnibus: | 124.288 | Durbin-Watson: | 0.945 |
---|---|---|---|
Prob(Omnibus): | 0.000 | Jarque-Bera (JB): | 244.026 |
Skew: | 1.362 | Prob(JB): | 1.02e-53 |
Kurtosis: | 5.038 | Cond. No. | 201. |
Notes:
[1] Standard Errors assume that the covariance matrix of the errors is correctly specified.
import statsmodels.formula.api as smf # R-style formula api
# ols model with intercept
ols_smf = smf.ols(formula='medv ~ lstat + age', data=boston)
# fitted model and summary
ols_smf_results = ols_smf.fit()
ols_smf_results.summary()
Dep. Variable: | medv | R-squared: | 0.551 |
---|---|---|---|
Model: | OLS | Adj. R-squared: | 0.549 |
Method: | Least Squares | F-statistic: | 309.0 |
Date: | Sat, 11 Nov 2023 | Prob (F-statistic): | 2.98e-88 |
Time: | 11:47:25 | Log-Likelihood: | -1637.5 |
No. Observations: | 506 | AIC: | 3281. |
Df Residuals: | 503 | BIC: | 3294. |
Df Model: | 2 | ||
Covariance Type: | nonrobust |
coef | std err | t | P>|t| | [0.025 | 0.975] | |
---|---|---|---|---|---|---|
Intercept | 33.2228 | 0.731 | 45.458 | 0.000 | 31.787 | 34.659 |
lstat | -1.0321 | 0.048 | -21.416 | 0.000 | -1.127 | -0.937 |
age | 0.0345 | 0.012 | 2.826 | 0.005 | 0.011 | 0.059 |
Omnibus: | 124.288 | Durbin-Watson: | 0.945 |
---|---|---|---|
Prob(Omnibus): | 0.000 | Jarque-Bera (JB): | 244.026 |
Skew: | 1.362 | Prob(JB): | 1.02e-53 |
Kurtosis: | 5.038 | Cond. No. | 201. |
Notes:
[1] Standard Errors assume that the covariance matrix of the errors is correctly specified.
def ols_formula(df, dependent_var, *excluded_cols):
'''
Generates the R style formula for statsmodels (patsy) given
the dataframe, dependent variable and optional excluded columns
as strings
'''
df_columns = list(df.columns.values)
df_columns.remove(dependent_var)
for col in excluded_cols:
df_columns.remove(col)
return dependent_var + ' ~ ' + ' + '.join(df_columns)
# ols model with intercept
ols_smf = smf.ols(formula=ols_formula(boston, 'medv'), data=boston)
# fitted model and summary
ols_smf_results = ols_smf.fit()
ols_smf_results.summary()
Dep. Variable: | medv | R-squared: | 0.741 |
---|---|---|---|
Model: | OLS | Adj. R-squared: | 0.734 |
Method: | Least Squares | F-statistic: | 108.1 |
Date: | Sat, 11 Nov 2023 | Prob (F-statistic): | 6.72e-135 |
Time: | 11:47:25 | Log-Likelihood: | -1498.8 |
No. Observations: | 506 | AIC: | 3026. |
Df Residuals: | 492 | BIC: | 3085. |
Df Model: | 13 | ||
Covariance Type: | nonrobust |
coef | std err | t | P>|t| | [0.025 | 0.975] | |
---|---|---|---|---|---|---|
Intercept | 36.4595 | 5.103 | 7.144 | 0.000 | 26.432 | 46.487 |
crim | -0.1080 | 0.033 | -3.287 | 0.001 | -0.173 | -0.043 |
zn | 0.0464 | 0.014 | 3.382 | 0.001 | 0.019 | 0.073 |
indus | 0.0206 | 0.061 | 0.334 | 0.738 | -0.100 | 0.141 |
chas | 2.6867 | 0.862 | 3.118 | 0.002 | 0.994 | 4.380 |
nox | -17.7666 | 3.820 | -4.651 | 0.000 | -25.272 | -10.262 |
rm | 3.8099 | 0.418 | 9.116 | 0.000 | 2.989 | 4.631 |
age | 0.0007 | 0.013 | 0.052 | 0.958 | -0.025 | 0.027 |
dis | -1.4756 | 0.199 | -7.398 | 0.000 | -1.867 | -1.084 |
rad | 0.3060 | 0.066 | 4.613 | 0.000 | 0.176 | 0.436 |
tax | -0.0123 | 0.004 | -3.280 | 0.001 | -0.020 | -0.005 |
ptratio | -0.9527 | 0.131 | -7.283 | 0.000 | -1.210 | -0.696 |
black | 0.0093 | 0.003 | 3.467 | 0.001 | 0.004 | 0.015 |
lstat | -0.5248 | 0.051 | -10.347 | 0.000 | -0.624 | -0.425 |
Omnibus: | 178.041 | Durbin-Watson: | 1.078 |
---|---|---|---|
Prob(Omnibus): | 0.000 | Jarque-Bera (JB): | 783.126 |
Skew: | 1.521 | Prob(JB): | 8.84e-171 |
Kurtosis: | 8.281 | Cond. No. | 1.51e+04 |
Notes:
[1] Standard Errors assume that the covariance matrix of the errors is correctly specified.
[2] The condition number is large, 1.51e+04. This might indicate that there are
strong multicollinearity or other numerical problems.
# variance inflation factors
from statsmodels.stats.outliers_influence import variance_inflation_factor as vif
# don't forget to add constant if the ols model includes intercept
boston_exog = sm.add_constant(boston.drop('medv', axis=1))
# too fancy for printing results?
for i, col in enumerate(boston_exog.columns):
if col == 'const':
pass
elif len(col) > 6:
print(col, ':', "{0:.2f}".format(vif(boston_exog.values, i)))
else:
print(col, '\t:', "{0:.2f}".format(vif(boston_exog.values, i)))
crim : 1.79
zn : 2.30
indus : 3.99
chas : 1.07
nox : 4.39
rm : 1.93
age : 3.10
dis : 3.96
rad : 7.48
tax : 9.01
ptratio : 1.80
black : 1.35
lstat : 2.94
# ols model with intercept
ols_smf = smf.ols(formula=ols_formula(boston, 'medv', 'age'), data=boston)
# fitted model and summary
ols_smf_results = ols_smf.fit()
ols_smf_results.summary()
Dep. Variable: | medv | R-squared: | 0.741 |
---|---|---|---|
Model: | OLS | Adj. R-squared: | 0.734 |
Method: | Least Squares | F-statistic: | 117.3 |
Date: | Sat, 11 Nov 2023 | Prob (F-statistic): | 6.08e-136 |
Time: | 11:47:25 | Log-Likelihood: | -1498.8 |
No. Observations: | 506 | AIC: | 3024. |
Df Residuals: | 493 | BIC: | 3079. |
Df Model: | 12 | ||
Covariance Type: | nonrobust |
coef | std err | t | P>|t| | [0.025 | 0.975] | |
---|---|---|---|---|---|---|
Intercept | 36.4369 | 5.080 | 7.172 | 0.000 | 26.456 | 46.418 |
crim | -0.1080 | 0.033 | -3.290 | 0.001 | -0.173 | -0.043 |
zn | 0.0463 | 0.014 | 3.404 | 0.001 | 0.020 | 0.073 |
indus | 0.0206 | 0.061 | 0.335 | 0.738 | -0.100 | 0.141 |
chas | 2.6890 | 0.860 | 3.128 | 0.002 | 1.000 | 4.378 |
nox | -17.7135 | 3.679 | -4.814 | 0.000 | -24.943 | -10.484 |
rm | 3.8144 | 0.408 | 9.338 | 0.000 | 3.012 | 4.617 |
dis | -1.4786 | 0.191 | -7.757 | 0.000 | -1.853 | -1.104 |
rad | 0.3058 | 0.066 | 4.627 | 0.000 | 0.176 | 0.436 |
tax | -0.0123 | 0.004 | -3.283 | 0.001 | -0.020 | -0.005 |
ptratio | -0.9522 | 0.130 | -7.308 | 0.000 | -1.208 | -0.696 |
black | 0.0093 | 0.003 | 3.481 | 0.001 | 0.004 | 0.015 |
lstat | -0.5239 | 0.048 | -10.999 | 0.000 | -0.617 | -0.430 |
Omnibus: | 178.343 | Durbin-Watson: | 1.078 |
---|---|---|---|
Prob(Omnibus): | 0.000 | Jarque-Bera (JB): | 786.386 |
Skew: | 1.523 | Prob(JB): | 1.73e-171 |
Kurtosis: | 8.294 | Cond. No. | 1.48e+04 |
Notes:
[1] Standard Errors assume that the covariance matrix of the errors is correctly specified.
[2] The condition number is large, 1.48e+04. This might indicate that there are
strong multicollinearity or other numerical problems.
3.6.4 Interaction Terms#
# ols model with intercept
ols_smf = smf.ols(formula='medv ~ lstat * age', data=boston)
# fitted model and summary
ols_smf_results = ols_smf.fit()
ols_smf_results.summary()
Dep. Variable: | medv | R-squared: | 0.556 |
---|---|---|---|
Model: | OLS | Adj. R-squared: | 0.553 |
Method: | Least Squares | F-statistic: | 209.3 |
Date: | Sat, 11 Nov 2023 | Prob (F-statistic): | 4.86e-88 |
Time: | 11:47:25 | Log-Likelihood: | -1635.0 |
No. Observations: | 506 | AIC: | 3278. |
Df Residuals: | 502 | BIC: | 3295. |
Df Model: | 3 | ||
Covariance Type: | nonrobust |
coef | std err | t | P>|t| | [0.025 | 0.975] | |
---|---|---|---|---|---|---|
Intercept | 36.0885 | 1.470 | 24.553 | 0.000 | 33.201 | 38.976 |
lstat | -1.3921 | 0.167 | -8.313 | 0.000 | -1.721 | -1.063 |
age | -0.0007 | 0.020 | -0.036 | 0.971 | -0.040 | 0.038 |
lstat:age | 0.0042 | 0.002 | 2.244 | 0.025 | 0.001 | 0.008 |
Omnibus: | 135.601 | Durbin-Watson: | 0.965 |
---|---|---|---|
Prob(Omnibus): | 0.000 | Jarque-Bera (JB): | 296.955 |
Skew: | 1.417 | Prob(JB): | 3.29e-65 |
Kurtosis: | 5.461 | Cond. No. | 6.88e+03 |
Notes:
[1] Standard Errors assume that the covariance matrix of the errors is correctly specified.
[2] The condition number is large, 6.88e+03. This might indicate that there are
strong multicollinearity or other numerical problems.
3.6.5 Non-linear Transformations of Predictors#
# ols model with intercept
ols_smf = smf.ols(formula='medv ~ lstat + np.power(lstat, 2)', data=boston)
# fitted model and summary
ols_smf_results = ols_smf.fit()
ols_smf_results.summary()
Dep. Variable: | medv | R-squared: | 0.641 |
---|---|---|---|
Model: | OLS | Adj. R-squared: | 0.639 |
Method: | Least Squares | F-statistic: | 448.5 |
Date: | Sat, 11 Nov 2023 | Prob (F-statistic): | 1.56e-112 |
Time: | 11:47:25 | Log-Likelihood: | -1581.3 |
No. Observations: | 506 | AIC: | 3169. |
Df Residuals: | 503 | BIC: | 3181. |
Df Model: | 2 | ||
Covariance Type: | nonrobust |
coef | std err | t | P>|t| | [0.025 | 0.975] | |
---|---|---|---|---|---|---|
Intercept | 42.8620 | 0.872 | 49.149 | 0.000 | 41.149 | 44.575 |
lstat | -2.3328 | 0.124 | -18.843 | 0.000 | -2.576 | -2.090 |
np.power(lstat, 2) | 0.0435 | 0.004 | 11.628 | 0.000 | 0.036 | 0.051 |
Omnibus: | 107.006 | Durbin-Watson: | 0.921 |
---|---|---|---|
Prob(Omnibus): | 0.000 | Jarque-Bera (JB): | 228.388 |
Skew: | 1.128 | Prob(JB): | 2.55e-50 |
Kurtosis: | 5.397 | Cond. No. | 1.13e+03 |
Notes:
[1] Standard Errors assume that the covariance matrix of the errors is correctly specified.
[2] The condition number is large, 1.13e+03. This might indicate that there are
strong multicollinearity or other numerical problems.
# anova of the two models
ols_smf = smf.ols(formula='medv ~ lstat', data=boston).fit()
ols_smf2 = smf.ols(formula='medv ~ lstat + np.power(lstat, 2)', data=boston).fit()
sm.stats.anova_lm(ols_smf, ols_smf2)
df_resid | ssr | df_diff | ss_diff | F | Pr(>F) | |
---|---|---|---|---|---|---|
0 | 504.00 | 19,472.38 | 0.00 | NaN | NaN | NaN |
1 | 503.00 | 15,347.24 | 1.00 | 4,125.14 | 135.20 | 0.00 |
f, axes = plt.subplots(4, 2, sharex=False, sharey=False)
f.set_figheight(20)
for axis, order in ((axes[0,0], 1), (axes[0,1], 2), (axes[1,0], 3), (axes[1,1], 5)):
sns.regplot('lstat', 'medv', data=boston, ax=axis, order=order, line_kws={'color': 'gray'}, scatter_kws={'alpha': 0.5})
for axis, order in ((axes[2,0], 1), (axes[2,1], 2), (axes[3,0], 3), (axes[3,1], 5)):
sns.residplot('lstat', 'medv', data=boston, ax=axis, order=order, line_kws={'color': 'gray'}, scatter_kws={'alpha': 0.5})
---------------------------------------------------------------------------
TypeError Traceback (most recent call last)
Cell In[20], line 5
2 f.set_figheight(20)
4 for axis, order in ((axes[0,0], 1), (axes[0,1], 2), (axes[1,0], 3), (axes[1,1], 5)):
----> 5 sns.regplot('lstat', 'medv', data=boston, ax=axis, order=order, line_kws={'color': 'gray'}, scatter_kws={'alpha': 0.5})
7 for axis, order in ((axes[2,0], 1), (axes[2,1], 2), (axes[3,0], 3), (axes[3,1], 5)):
8 sns.residplot('lstat', 'medv', data=boston, ax=axis, order=order, line_kws={'color': 'gray'}, scatter_kws={'alpha': 0.5})
TypeError: regplot() got multiple values for argument 'data'
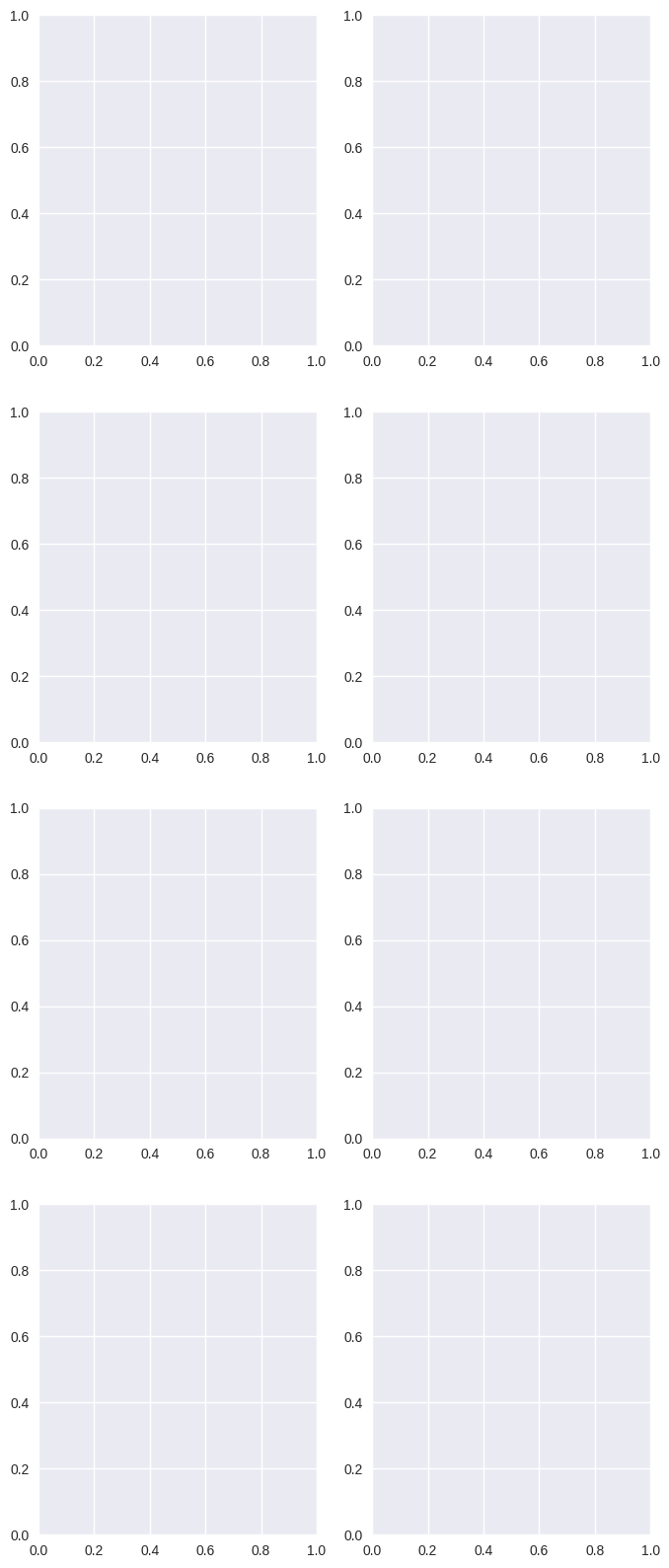
# polynomial ols model with intercept
# numpy way
#ols_smf = smf.ols(formula='medv ~ lstat + np.power(lstat, 2) + np.power(lstat, 3) + np.power(lstat, 4) + np.power(lstat, 5)',
# data=boston)
# patsy way
ols_smf = smf.ols(formula='medv ~ lstat + I(lstat**2) + I(lstat**3) + I(lstat**4) + I(lstat**5)',
data=boston)
# fitted model and summary
ols_smf_results = ols_smf.fit()
ols_smf_results.summary()
Dep. Variable: | medv | R-squared: | 0.682 |
---|---|---|---|
Model: | OLS | Adj. R-squared: | 0.679 |
Method: | Least Squares | F-statistic: | 214.2 |
Date: | Sat, 11 Nov 2023 | Prob (F-statistic): | 8.73e-122 |
Time: | 11:47:26 | Log-Likelihood: | -1550.6 |
No. Observations: | 506 | AIC: | 3113. |
Df Residuals: | 500 | BIC: | 3139. |
Df Model: | 5 | ||
Covariance Type: | nonrobust |
coef | std err | t | P>|t| | [0.025 | 0.975] | |
---|---|---|---|---|---|---|
Intercept | 67.6997 | 3.604 | 18.783 | 0.000 | 60.618 | 74.781 |
lstat | -11.9911 | 1.526 | -7.859 | 0.000 | -14.989 | -8.994 |
I(lstat ** 2) | 1.2728 | 0.223 | 5.703 | 0.000 | 0.834 | 1.711 |
I(lstat ** 3) | -0.0683 | 0.014 | -4.747 | 0.000 | -0.097 | -0.040 |
I(lstat ** 4) | 0.0017 | 0.000 | 4.143 | 0.000 | 0.001 | 0.003 |
I(lstat ** 5) | -1.632e-05 | 4.42e-06 | -3.692 | 0.000 | -2.5e-05 | -7.63e-06 |
Omnibus: | 144.085 | Durbin-Watson: | 0.987 |
---|---|---|---|
Prob(Omnibus): | 0.000 | Jarque-Bera (JB): | 494.545 |
Skew: | 1.292 | Prob(JB): | 4.08e-108 |
Kurtosis: | 7.096 | Cond. No. | 1.37e+08 |
Notes:
[1] Standard Errors assume that the covariance matrix of the errors is correctly specified.
[2] The condition number is large, 1.37e+08. This might indicate that there are
strong multicollinearity or other numerical problems.
# polynomial ols model with intercept
ols_smf = smf.ols(formula='medv ~ np.log(rm)', data=boston)
# fitted model and summary
ols_smf_results = ols_smf.fit()
ols_smf_results.summary()
Dep. Variable: | medv | R-squared: | 0.436 |
---|---|---|---|
Model: | OLS | Adj. R-squared: | 0.435 |
Method: | Least Squares | F-statistic: | 389.3 |
Date: | Sat, 11 Nov 2023 | Prob (F-statistic): | 1.22e-64 |
Time: | 11:47:26 | Log-Likelihood: | -1695.4 |
No. Observations: | 506 | AIC: | 3395. |
Df Residuals: | 504 | BIC: | 3403. |
Df Model: | 1 | ||
Covariance Type: | nonrobust |
coef | std err | t | P>|t| | [0.025 | 0.975] | |
---|---|---|---|---|---|---|
Intercept | -76.4878 | 5.028 | -15.213 | 0.000 | -86.366 | -66.610 |
np.log(rm) | 54.0546 | 2.739 | 19.732 | 0.000 | 48.672 | 59.437 |
Omnibus: | 117.102 | Durbin-Watson: | 0.681 |
---|---|---|---|
Prob(Omnibus): | 0.000 | Jarque-Bera (JB): | 584.336 |
Skew: | 0.916 | Prob(JB): | 1.30e-127 |
Kurtosis: | 7.936 | Cond. No. | 38.9 |
Notes:
[1] Standard Errors assume that the covariance matrix of the errors is correctly specified.
sns.regplot('rm',
'medv',
data=boston,
logx=True,
line_kws={'color': 'gray'},
scatter_kws={'alpha': 0.5});
---------------------------------------------------------------------------
TypeError Traceback (most recent call last)
Cell In[23], line 1
----> 1 sns.regplot('rm',
2 'medv',
3 data=boston,
4 logx=True,
5 line_kws={'color': 'gray'},
6 scatter_kws={'alpha': 0.5});
TypeError: regplot() got multiple values for argument 'data'
3.6.6 Qualitative Predictors#
carseats = pd.read_csv('../datasets/Carseats.csv', index_col=0)
carseats
Sales | CompPrice | Income | Advertising | Population | Price | ShelveLoc | Age | Education | Urban | US | |
---|---|---|---|---|---|---|---|---|---|---|---|
1 | 9.50 | 138 | 73 | 11 | 276 | 120 | Bad | 42 | 17 | Yes | Yes |
2 | 11.22 | 111 | 48 | 16 | 260 | 83 | Good | 65 | 10 | Yes | Yes |
3 | 10.06 | 113 | 35 | 10 | 269 | 80 | Medium | 59 | 12 | Yes | Yes |
4 | 7.40 | 117 | 100 | 4 | 466 | 97 | Medium | 55 | 14 | Yes | Yes |
5 | 4.15 | 141 | 64 | 3 | 340 | 128 | Bad | 38 | 13 | Yes | No |
... | ... | ... | ... | ... | ... | ... | ... | ... | ... | ... | ... |
396 | 12.57 | 138 | 108 | 17 | 203 | 128 | Good | 33 | 14 | Yes | Yes |
397 | 6.14 | 139 | 23 | 3 | 37 | 120 | Medium | 55 | 11 | No | Yes |
398 | 7.41 | 162 | 26 | 12 | 368 | 159 | Medium | 40 | 18 | Yes | Yes |
399 | 5.94 | 100 | 79 | 7 | 284 | 95 | Bad | 50 | 12 | Yes | Yes |
400 | 9.71 | 134 | 37 | 0 | 27 | 120 | Good | 49 | 16 | Yes | Yes |
400 rows × 11 columns
# ols model with intercept
form = ols_formula(carseats, 'Sales', 'ShelveLoc') + ' + Income:Advertising + Price:Age + C(ShelveLoc)'
ols_smf = smf.ols(formula=form, data=carseats)
# fitted model and summary
ols_smf_results = ols_smf.fit()
ols_smf_results.summary()
Dep. Variable: | Sales | R-squared: | 0.876 |
---|---|---|---|
Model: | OLS | Adj. R-squared: | 0.872 |
Method: | Least Squares | F-statistic: | 210.0 |
Date: | Sat, 11 Nov 2023 | Prob (F-statistic): | 6.14e-166 |
Time: | 11:47:26 | Log-Likelihood: | -564.67 |
No. Observations: | 400 | AIC: | 1157. |
Df Residuals: | 386 | BIC: | 1213. |
Df Model: | 13 | ||
Covariance Type: | nonrobust |
coef | std err | t | P>|t| | [0.025 | 0.975] | |
---|---|---|---|---|---|---|
Intercept | 6.5756 | 1.009 | 6.519 | 0.000 | 4.592 | 8.559 |
Urban[T.Yes] | 0.1402 | 0.112 | 1.247 | 0.213 | -0.081 | 0.361 |
US[T.Yes] | -0.1576 | 0.149 | -1.058 | 0.291 | -0.450 | 0.135 |
C(ShelveLoc)[T.Good] | 4.8487 | 0.153 | 31.724 | 0.000 | 4.548 | 5.149 |
C(ShelveLoc)[T.Medium] | 1.9533 | 0.126 | 15.531 | 0.000 | 1.706 | 2.201 |
CompPrice | 0.0929 | 0.004 | 22.567 | 0.000 | 0.085 | 0.101 |
Income | 0.0109 | 0.003 | 4.183 | 0.000 | 0.006 | 0.016 |
Advertising | 0.0702 | 0.023 | 3.107 | 0.002 | 0.026 | 0.115 |
Population | 0.0002 | 0.000 | 0.433 | 0.665 | -0.001 | 0.001 |
Price | -0.1008 | 0.007 | -13.549 | 0.000 | -0.115 | -0.086 |
Age | -0.0579 | 0.016 | -3.633 | 0.000 | -0.089 | -0.027 |
Education | -0.0209 | 0.020 | -1.063 | 0.288 | -0.059 | 0.018 |
Income:Advertising | 0.0008 | 0.000 | 2.698 | 0.007 | 0.000 | 0.001 |
Price:Age | 0.0001 | 0.000 | 0.801 | 0.424 | -0.000 | 0.000 |
Omnibus: | 1.281 | Durbin-Watson: | 2.047 |
---|---|---|---|
Prob(Omnibus): | 0.527 | Jarque-Bera (JB): | 1.147 |
Skew: | 0.129 | Prob(JB): | 0.564 |
Kurtosis: | 3.050 | Cond. No. | 1.31e+05 |
Notes:
[1] Standard Errors assume that the covariance matrix of the errors is correctly specified.
[2] The condition number is large, 1.31e+05. This might indicate that there are
strong multicollinearity or other numerical problems.
from patsy.contrasts import Treatment
Treatment(reference=0).code_without_intercept(list(carseats['ShelveLoc'].unique())).matrix
array([[0., 0.],
[1., 0.],
[0., 1.]])
3.6.7 Writing Functions#
come on…